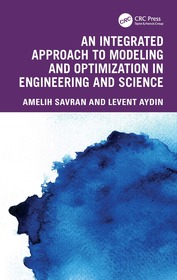
ISBN13: | 9781032782799 |
ISBN10: | 103278279X |
Binding: | Hardback |
No. of pages: | 343 pages |
Size: | 229x152 mm |
Weight: | 453 g |
Language: | English |
Illustrations: | 45 Illustrations, black & white; 1 Halftones, black & white; 44 Line drawings, black & white; 85 Tables, black & white |
683 |
An Integrated Approach to Modeling and Optimization in Engineering and Science
GBP 76.99
Click here to subscribe.
Not in stock at Prospero.
An Integrated Approach to Modeling and Optimization in Engineering and Science is a technical book written with the aim to evaluate the modeling and design processes of engineering systems with an integrated approach.
An Integrated Approach to Modeling and Optimization in Engineering and Science examines the effects of experimental design, mathematical modeling, and optimization processes for solving many different problems. The Experimental Design Method, Central Composite, Full Factorial, Taguchi, Box-Behnken, and D-Optimal methods are used, and the effects of the datasets obtained by these methods on mathematical modeling are investigated.
This book will help graduates and senior undergraduates in courses on experimental design, modeling, optimization, and interdisciplinary engineering studies. It will also be of interest to research and development engineers and professionals working in scientific institutions based on design, modeling, and optimization.
1. Introduction. 2. Design of Experiment, Mathematical Modeling, and Optimization. 3. Comparison of ANN and Neuro Regression Methods in Mathematical Modeling. 4. Evaluation of R2 as a Model Assessment Criterion. 5. Questioning the Adequacy of Using Polynomial Structures. 6. The Effect of Using the Taguchi Method in Experimental Design on Mathematical Modeling. 7. Comparison of Different Test and Validation Methods Used in Mathematical Modeling. 8. Comparison of Different Model Assessment Criteria Used in Mathematical Modeling. 9. Comparison of the Effects of Experimental Design Methods on Mathematical Modeling. 10. Special Functions in Mathematical Modeling. 11. Conclusion.