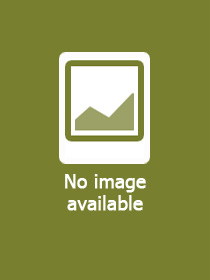
ISBN13: | 9780443235177 |
ISBN10: | 0443235171 |
Binding: | Paperback |
No. of pages: | 288 pages |
Size: | 229x152 mm |
Language: | English |
700 |
Artificial Intelligence for Subsurface Characterization and Monitoring
EUR 155.00
Click here to subscribe.
Artificial Intelligence for Subsurface Characterization and Monitoring provides an in-depth examination of how deep learning accelerates the process of subsurface characterization and monitoring and provides an end-to-end solution. In recent years, deep learning has been introduced to the geoscience community to overcome some longstanding technical challenges. This book explores some of the most important topics in this discipline to explain the unique capability of deep learning in subsurface characterization for hydrocarbon exploration and production and for energy transition. Readers will discover deep learning methods that can improve the quality and efficiency of many of the key steps in subsurface characterization and monitoring. The text is organized into five parts. The first two parts explore deep learning for data enrichment and well log data, including information extraction from unstructured well reports as well as log data QC and processing. Next is a review of deep learning applied to seismic data and data integration, which also covers intelligent processing for clearer seismic images and rock property inversion and validation. The closing section looks at deep learning in time lapse scenarios, including sparse data reconstruction for reducing the cost of 4D seismic data, time-lapse seismic data repeatability enforcement, and direct property prediction from pre-migration seismic data.
- Focus on deep learning applications for geoscience provides a one-stop reference for deep learning applications for geoscience.
- Comprehensive examples of the state-of-art techniques throughout the subsurface characterization workflow provide readers with a chance to familiarize themselves with deep learning applications not only in their own field of expertise but also in other relevant fields.
- All applications come with realistic field dataset examples so that readers can learn what to expect in real life.
1. Rejuvenating legacy data by digitizing raster logs
2. Information extraction from unstructured well reports
Part II Deep learning applied to well log data
3. Well log data QC and processing
4. Automatic well marker picking
5. Automatic log interpretationPart III Deep learning applied to seismic data
6. Intelligent processing for clearer seismic images
7. Seismic interpretation with improved quality and efficiency
Part IV Deep learning for data integration
8. Automatic seismic-well tie
9. Rock property inversion and validation
Part V Deep learning in time lapse scenarios
10. Time-lapse seismic data repeatability enforcement
11. Direct property prediction from premigration seismic data