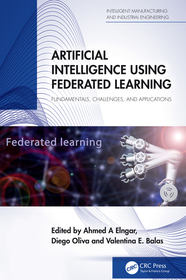
ISBN13: | 9781032771649 |
ISBN10: | 103277164X |
Binding: | Hardback |
No. of pages: | 344 pages |
Size: | 234x156 mm |
Language: | English |
Illustrations: | 93 Illustrations, black & white; 9 Halftones, black & white; 84 Line drawings, black & white; 37 Tables, black & white |
700 |
Engineering in general
Electrical engineering and telecommunications, precision engineering
Energy industry
Engineering sciences
System organization
Computer architecture, logic design
Database management softwares
Artificial Intelligence
Privacy, data security
Safety and health aspects of computing
Environmental sciences
Internet in general
Engineering in general (charity campaign)
Electrical engineering and telecommunications, precision engineering (charity campaign)
Energy industry (charity campaign)
Engineering sciences (charity campaign)
System organization (charity campaign)
Computer architecture, logic design (charity campaign)
Database management softwares (charity campaign)
Artificial Intelligence (charity campaign)
Privacy, data security (charity campaign)
Safety and health aspects of computing (charity campaign)
Environmental sciences (charity campaign)
Internet in general (charity campaign)
Artificial Intelligence Using Federated Learning
GBP 130.00
Click here to subscribe.
Federated machine learning is a novel approach to combining distributed machine learning, cryptography, security, and incentive mechanism design. It allows organizations to keep sensitive and private data on users or customers decentralized and secure, helping them comply with stringent data protection regulations like GDPR and CCPA.
Federated machine learning is a novel approach to combining distributed machine learning, cryptography, security, and incentive mechanism design. It allows organizations to keep sensitive and private data on users or customers decentralized and secure, helping them comply with stringent data protection regulations like GDPR and CCPA.
Artificial Intelligence Using Federated Learning: Fundamentals, Challenges, and Applications enables training AI models on a large number of decentralized devices or servers, making it a scalable and efficient solution. It also allows organizations to create more versatile AI models by training them on data from diverse sources or domains. This approach can unlock innovative use cases in fields like healthcare, finance, and IoT, where data privacy is paramount.
The book is designed for researchers working in Intelligent Federated Learning and its related applications, as well as technology development, and is also of interest to academicians, data scientists, industrial professionals, researchers, and students.
1. Federated Learning: Overview, Challenges and Ethical Considerations. 2. In-depth Analysis of Artificial Intelligence Practices: Robot Tutors and Federated Learning Approach in English Education. 3. Enabling Federated Learning in the Classroom: Sociotechnical Ecosystem on Artificial Intelligence Integration in Educational Practices. 4. Real-Time Implementation of Improved Automatic Number Plate Recognition Using Federated Learning. 5. Fake Currency Identification Using Artificial Intelligence and Federated Learning. 6. Blockchain-Enhanced Federated Learning for Privacy-Preserving Collaboration. 7. Federated Learning-based Smart Transportation Solutions: Deploying Lightweight Models on Edge Devices in the Internet of Vehicle. 8. Application of Artificial Intelligence (AI) and Federated Learning (FL) in Petroleum Processing. 9. Artificial Intelligence Using Federated Learning. 10. Applications of Federated Learning in AI, IoT, Healthcare, Finance, Banking and Cross-Domain Learning. 11. Exploring Future Trends and Emerging Applications: A Glimpse into Tomorrow's Landscape. 12. Securing Federated Deep Learning: Privacy Risks and Countermeasures. 13. IoT Networks: Integrated Learning For Privacy-Preserving Machine Learning. 14. Federated Query Processing for Data Integration using Semantic Web Technologies: A Review.