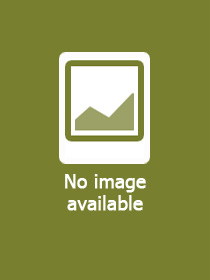
Computational Methods for Time-Series Analysis in Earth Sciences
- Publisher's listprice EUR 158.99
-
The price is estimated because at the time of ordering we do not know what conversion rates will apply to HUF / product currency when the book arrives. In case HUF is weaker, the price increases slightly, in case HUF is stronger, the price goes lower slightly.
- Discount 10% (cc. 6 744 Ft off)
- Discounted price 60 698 Ft (57 808 Ft + 5% VAT)
67 443 Ft
Availability
Not yet published.
Why don't you give exact delivery time?
Delivery time is estimated on our previous experiences. We give estimations only, because we order from outside Hungary, and the delivery time mainly depends on how quickly the publisher supplies the book. Faster or slower deliveries both happen, but we do our best to supply as quickly as possible.
Product details:
- Publisher Elsevier
- Date of Publication 10 June 2025
- ISBN 9780443336317
- Binding Paperback
- No. of pages420 pages
- Size 229x152 mm
- Weight 450 g
- Language English 700
Categories
Long description:
Computational Methods for Time-Series Analysis in Earth Sciences bridges the gap between theoretical knowledge and practical application, offering a deep dive into the utilization of R programming for managing, analyzing, and forecasting time-series data within the Earth sciences. The book systematically unfolds the layers of data manipulation, graphical representation, and sampling to prepare the reader for complex analyses and predictive modeling, from the basics of signal processing to the nuances of machine learning. It presents cutting-edge techniques, such as neural networks, kernel-based methods, and evolutionary algorithms, specifically tailored to tackle challenges, and provides practical case studies to aid readers.
This is a valuable resource for scientists, researchers, and students delving into the intricacies of Earth's environmental patterns and cycles through the lens of computational analysis. It guides readers through various computational approaches for deciphering spatial and temporal data.
- Focuses on the use of R for time series analysis and the application of these methods directly to Earth and environmental datasets
- Integrates Machine Learning techniques, enabling readers to explore advanced computational methods for forecasting and modeling
- Includes case studies with real-world applications, providing readers with examples on how to translate computational skills into tangible outcomes
Table of Contents:
Section 1: Theory and Computational Methods
1. Introduction to R: Data manipulation, graphics, and sampling
2. Time series analysis for earth sciences with R
3. Signal processing with R for earth sciences.
4. Spatial Analyses with R for earth sciences
5. Deterministic modelling with R for earth sciences
6. Machine learning with R for earth sciences
Section 2: Case of Studies and Applications
7. Predicting Sandy Soils' Hydraulic Properties and Drainage Capacities with Neural Networks
8. Prognostication of Real-Time Hourly Precipitation using Kernel-based Techniques
9. Integrating Upstream Runoff and Local Rainfall for Real-Time Flood Prediction
10. Pre-diagnosis of Flooding Using Real-Time Monitoring of Climate Parameters
11. Comparing Local vs. External Data Analysis for Forecasting
12. Evolutionary Kernel Extreme Learning Machine for Real-Time Forecasting
13. A Stochastic AI Method for Predicting Climatic Variables' Spatio-Temporal Changes Under Future Climates - Data Preparation and Preprocessing
14. A Novel AI Stochastic Approach for Predicting Spatio-Temporal Variables and Changes Under Future Climate Conditions: Google Earth Engine's Benefits and Challenges; An Intro to SOILPARAM APP
15. A Novel AI Stochastic Method for Predicting Changes in Space and Time: Linear Modeling
16. A Novel AI Stochastic Method for Predicting Changes: Nonlinear Modeling
17. A Combination of Satellite Observations and Machine Learning Technique for Terrestrial Anomaly Estimation