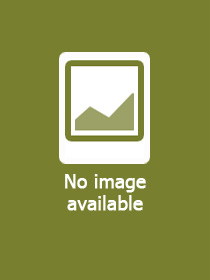
ISBN13: | 9781032978079 |
ISBN10: | 1032978074 |
Binding: | Hardback |
No. of pages: | 104 pages |
Size: | 234x156 mm |
Language: | English |
Illustrations: | 9 Illustrations, black & white; 9 Line drawings, black & white; 8 Tables, black & white |
700 |
Electrical engineering and telecommunications, precision engineering
Theory of computing, computing in general
Data management in computer systems
Computer architecture, logic design
Supercomputers
Operating systems and graphical user interfaces
Computer programming in general
Database management softwares
Applied linguistics
Concept Drift in Large Language Models
GBP 79.99
Click here to subscribe.
This book explores the application of the complex relationship between concept drift and cutting-edge large language models to address the problems and opportunities in navigating changing data landscapes.
This book explores the application of the complex relationship between concept drift and cutting-edge large language models to address the problems and opportunities in navigating changing data landscapes. It discusses the theoretical basis of concept drift and its consequences for large language models, particularly the transformative power of cutting-edge models such as GPT-3.5 and GPT-4. It offers real-world case studies to observe firsthand how concept drift influences the performance of language models in a variety of circumstances, delivering valuable lessons learnt and actionable takeaways. The book is designed for professionals, AI practitioners, and scholars, focused on natural language processing, machine learning, and artificial intelligence.
- Examines concept drift in AI, particularly its impact on large language models
- Analyses how concept drift affects large language models and its theoretical and practical consequences
- Covers detection methods and practical implementation challenges in language models
- Showcases examples of concept drift in GPT models and lessons learnt from their performance
- Identifies future research avenues and recommendations for practitioners tackling concept drift in large language models
1. Introduction 2. Concept Drift Fundamentals 3. Large Language Models 4. Concept Drift and Large Language Models 5. Detecting Concept Drift in Language Models 6. Adapting Language Models 7. Natural Language Processing 8. Limitations and Challenges 9. Conclusion and Future Directions