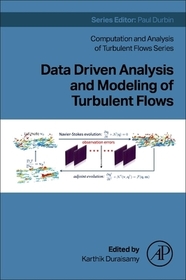
Product details:
ISBN13: | 9780323950435 |
ISBN10: | 0323950434 |
Binding: | Paperback |
No. of pages: | 414 pages |
Size: | 229x152 mm |
Language: | English |
700 |
Category:
Data Driven Analysis and Modeling of Turbulent Flows
Publisher: Academic Press
Date of Publication: 7 March 2025
Normal price:
Publisher's listprice:
EUR 195.00
EUR 195.00
Your price:
76 290 (72 657 HUF + 5% VAT )
discount is: 10% (approx 8 477 HUF off)
The discount is only available for 'Alert of Favourite Topics' newsletter recipients.
Click here to subscribe.
Click here to subscribe.
Availability:
Not yet published.
Long description:
Data-driven Analysis and Modeling of Turbulent Flows explains methods for the analysis of large fields of data, and uncovering models and model improvements from numerical or experimental data on turbulence.
Turbulence simulations generate large data sets, and the extraction of useful information from these data fields is an important and challenging task. Statistical learning and machine learning have provided many ways of helping, and this book explains how to use such methods for extracting, treating, and optimizing data to improve predictive turbulence models. These include methods such as POD, SPOD and DMD, for the extraction of modes peculiar to the data, as well as several reduced order models.
This resource is essential reading for those developing turbulence models, performing turbulence simulations or interpreting turbulence simulation results.
Turbulence simulations generate large data sets, and the extraction of useful information from these data fields is an important and challenging task. Statistical learning and machine learning have provided many ways of helping, and this book explains how to use such methods for extracting, treating, and optimizing data to improve predictive turbulence models. These include methods such as POD, SPOD and DMD, for the extraction of modes peculiar to the data, as well as several reduced order models.
This resource is essential reading for those developing turbulence models, performing turbulence simulations or interpreting turbulence simulation results.
- Provides instructions for the most important statistical learning techniques
- Explains the basics of reduced order modeling
- Describes a wide range of data analysis methods evolving from linear and non-linear flow decomposition
Table of Contents:
1. A roadmap for data-driven analysis and modeling of turbulent flows
2. Modal decomposition: POD, SPOD, DMD
3. Statistical learning: Neural nets, sparse regression, Lasso
4. Resolvents
5. Projection-based Reduced Order Modeling
6. Data-assimilation and flow estimation
7. Data-driven control
8. Model-consistent inference and learning
9. Parameter estimation and uncertainty quantification
10. Stress representations
11. Evolutionary optimization
12. Emerging topics: Super resolution
2. Modal decomposition: POD, SPOD, DMD
3. Statistical learning: Neural nets, sparse regression, Lasso
4. Resolvents
5. Projection-based Reduced Order Modeling
6. Data-assimilation and flow estimation
7. Data-driven control
8. Model-consistent inference and learning
9. Parameter estimation and uncertainty quantification
10. Stress representations
11. Evolutionary optimization
12. Emerging topics: Super resolution