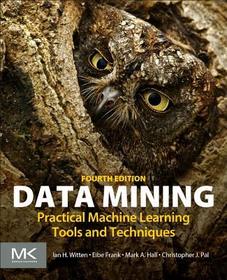
Data Mining
Practical Machine Learning Tools and Techniques
- Publisher's listprice EUR 58.95
-
The price is estimated because at the time of ordering we do not know what conversion rates will apply to HUF / product currency when the book arrives. In case HUF is weaker, the price increases slightly, in case HUF is stronger, the price goes lower slightly.
- Discount 10% (cc. 2 501 Ft off)
- Discounted price 22 505 Ft (21 434 Ft + 5% VAT)
25 006 Ft
Availability
Estimated delivery time: In stock at the publisher, but not at Prospero's office. Delivery time approx. 3-5 weeks.
Not in stock at Prospero.
Why don't you give exact delivery time?
Delivery time is estimated on our previous experiences. We give estimations only, because we order from outside Hungary, and the delivery time mainly depends on how quickly the publisher supplies the book. Faster or slower deliveries both happen, but we do our best to supply as quickly as possible.
Product details:
- Edition number 4
- Publisher Morgan Kaufmann
- Date of Publication 20 December 2016
- ISBN 9780128042915
- Binding Paperback
- No. of pages654 pages
- Size 235x191 mm
- Weight 1290 g
- Language English 200
Categories
Long description:
Data Mining: Practical Machine Learning Tools and Techniques, Fourth Edition, offers a thorough grounding in machine learning concepts, along with practical advice on applying these tools and techniques in real-world data mining situations. This highly anticipated fourth edition of the most acclaimed work on data mining and machine learning teaches readers everything they need to know to get going, from preparing inputs, interpreting outputs, evaluating results, to the algorithmic methods at the heart of successful data mining approaches.
Extensive updates reflect the technical changes and modernizations that have taken place in the field since the last edition, including substantial new chapters on probabilistic methods and on deep learning. Accompanying the book is a new version of the popular WEKA machine learning software from the University of Waikato. Authors Witten, Frank, Hall, and Pal include today's techniques coupled with the methods at the leading edge of contemporary research.
Please visit the book companion website at https://www.cs.waikato.ac.nz/~ml/weka/book.html.
It contains
- Powerpoint slides for Chapters 1-12. This is a very comprehensive teaching resource, with many PPT slides covering each chapter of the book
- Online Appendix on the Weka workbench; again a very comprehensive learning aid for the open source software that goes with the book
- Table of contents, highlighting the many new sections in the 4th edition, along with reviews of the 1st edition, errata, etc.
- Provides a thorough grounding in machine learning concepts, as well as practical advice on applying the tools and techniques to data mining projects
- Presents concrete tips and techniques for performance improvement that work by transforming the input or output in machine learning methods
- Includes a downloadable WEKA software toolkit, a comprehensive collection of machine learning algorithms for data mining tasks-in an easy-to-use interactive interface
- Includes open-access online courses that introduce practical applications of the material in the book
"...this volume is the most accessible introduction to data mining to appear in recent years. It is worthy of a fourth edition." --Computing Reviews
MoreTable of Contents:
Part I: Introduction to data mining
1. What's it all about?
2. Input: Concepts, instances, attributes
3. Output: Knowledge representation
4. Algorithms: The basic methods
5. Credibility: Evaluating what's been learned
Part II. More advanced machine learning schemes
6. Trees and rules
7. Extending instance-based and linear models
8. Data transformations
9. Probabilistic methods
10. Deep learning
11. Beyond supervised and unsupervised learning
12. Ensemble learning
13. Moving on: applications and beyond