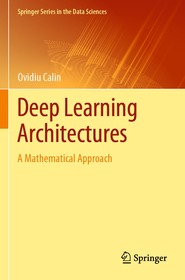
ISBN13: | 9783030367237 |
ISBN10: | 3030367231 |
Binding: | Paperback |
No. of pages: | 760 pages |
Size: | 254x178 mm |
Weight: | 1480 g |
Language: | English |
Illustrations: | 172 Illustrations, black & white; 35 Illustrations, color |
889 |
Applied mathematics
Theory of computing, computing in general
Further readings in mathematics
Springer Yellow Sale
Applied mathematics (charity campaign)
Theory of computing, computing in general (charity campaign)
Further readings in mathematics (charity campaign)
Springer Yellow Sale (charity campaign)
Deep Learning Architectures
EUR 69.54
Click here to subscribe.
Not in stock at Prospero.
This book describes how neural networks operate from the mathematical point of view. As a result, neural networks can be interpreted both as function universal approximators and information processors. The book bridges the gap between ideas and concepts of neural networks, which are used nowadays at an intuitive level, and the precise modern mathematical language, presenting the best practices of the former and enjoying the robustness and elegance of the latter.
This book can be used in a graduate course in deep learning, with the first few parts being accessible to senior undergraduates. In addition, the book will be of wide interest to machine learning researchers who are interested in a theoretical understanding of the subject.
This book describes how neural networks operate from the mathematical point of view. As a result, neural networks can be interpreted both as function universal approximators and information processors. The book bridges the gap between ideas and concepts of neural networks, which are used nowadays at an intuitive level, and the precise modern mathematical language, presenting the best practices of the former and enjoying the robustness and elegance of the latter.
This book can be used in a graduate course in deep learning, with the first few parts being accessible to senior undergraduates. In addition, the book will be of wide interest to machine learning researchers who are interested in a theoretical understanding of the subject.
?This book is useful to students who have already had an introductory course in machine learning and are further interested to deepen their understanding of the machine learning material from the mathematical point of view.? (T. C. Mohan, zbMATH 1441.68001, 2020)