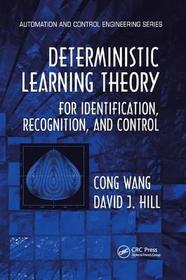
Deterministic Learning Theory for Identification, Recognition, and Control
For Identiflcation, Recognition, and Conirol
Series: Automation and Control Engineering;
- Publisher's listprice GBP 48.99
-
The price is estimated because at the time of ordering we do not know what conversion rates will apply to HUF / product currency when the book arrives. In case HUF is weaker, the price increases slightly, in case HUF is stronger, the price goes lower slightly.
- Discount 10% (cc. 2 479 Ft off)
- Discounted price 22 314 Ft (21 252 Ft + 5% VAT)
24 793 Ft
Availability
Estimated delivery time: In stock at the publisher, but not at Prospero's office. Delivery time approx. 3-5 weeks.
Not in stock at Prospero.
Why don't you give exact delivery time?
Delivery time is estimated on our previous experiences. We give estimations only, because we order from outside Hungary, and the delivery time mainly depends on how quickly the publisher supplies the book. Faster or slower deliveries both happen, but we do our best to supply as quickly as possible.
Product details:
- Edition number 1
- Publisher CRC Press
- Date of Publication 6 October 2017
- ISBN 9781138112056
- Binding Paperback
- No. of pages207 pages
- Size 234x156 mm
- Weight 453 g
- Language English
- Illustrations 147 Illustrations, black & white 0
Categories
Short description:
Offering a new perspective, this book provides systematic design approaches for the identification, control, and recognition of nonlinear systems in uncertain environments. It introduces the concepts of deterministic learning theory and then discusses the persistent excitation property of RBF networks. The authors describe the theory of deterministic learning processes and address dynamical pattern recognition and pattern-based control processes. They present a new model of dynamical parallel distributed processing applicable to the detection and isolation of oscillation faults, ECG/EEG pattern recognition, robot learning and control, and security analysis and control of power systems.
MoreLong description:
Deterministic Learning Theory for Identification, Recognition, and Control presents a unified conceptual framework for knowledge acquisition, representation, and knowledge utilization in uncertain dynamic environments. It provides systematic design approaches for identification, recognition, and control of linear uncertain systems. Unlike many books currently available that focus on statistical principles, this book stresses learning through closed-loop neural control, effective representation and recognition of temporal patterns in a deterministic way.
A Deterministic View of Learning in Dynamic Environments
The authors begin with an introduction to the concepts of deterministic learning theory, followed by a discussion of the persistent excitation property of RBF networks. They describe the elements of deterministic learning, and address dynamical pattern recognition and pattern-based control processes. The results are applicable to areas such as detection and isolation of oscillation faults, ECG/EEG pattern recognition, robot learning and control, and security analysis and control of power systems.
A New Model of Information Processing
This book elucidates a learning theory which is developed using concepts and tools from the discipline of systems and control. Fundamental knowledge about system dynamics is obtained from dynamical processes, and is then utilized to achieve rapid recognition of dynamical patterns and pattern-based closed-loop control via the so-called internal and dynamical matching of system dynamics. This actually represents a new model of information processing, i.e. a model of dynamical parallel distributed processing (DPDP).
MoreTable of Contents:
Introduction. RBF Networks and the PE Condition. Locally Accurate Identification of Nonlinear Systems. Learning from Closed-Loop Neural Control. Rapid Recognition of Dynamical Patterns. Deterministic Learning using Output Measurements. Applications of Deterministic Learning. Conclusions.
More