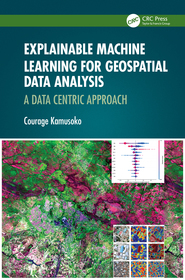
ISBN13: | 9781032503806 |
ISBN10: | 1032503807 |
Binding: | Hardback |
No. of pages: | 280 pages |
Size: | 234x156 mm |
Weight: | 453 g |
Language: | English |
Illustrations: | 69 Illustrations, black & white; 24 Illustrations, color; 11 Halftones, black & white; 8 Halftones, color; 58 Line drawings, black & white; 16 Line drawings, color; 10 Tables, black & white |
677 |
Explainable Machine Learning for Geospatial Data Analysis
GBP 110.00
Click here to subscribe.
Not in stock at Prospero.
This book highlights and explains the details of machine learning models used in geospatial data analysis. It demonstrates the need for a data-centric explainable machine learning approach for obtaining new insights from geospatial data analysis and how they are applied to solve various environmental problems from forestry to climate change.
Explainable machine learning (XML), a subfield of AI, is focused on making complex AI models understandable to humans. This book highlights and explains the details of machine learning models used in geospatial data analysis. It demonstrates the need for a data-centric, explainable machine learning approach to obtain new insights from geospatial data. It presents the opportunities, challenges, and gaps in the machine and deep learning approaches for geospatial data analysis and how they are applied to solve various environmental problems in land cover changes and in modeling forest canopy height and aboveground biomass density. The author also includes guidelines and code scripts (R, Python) valuable for practical readers.
Features
- Data-centric explainable machine learning (ML) approaches for geospatial data analysis.
- The foundations and approaches to explainable ML and deep learning.
- Several case studies from urban land cover and forestry where existing explainable machine learning methods are applied.
- Descriptions of the opportunities, challenges, and gaps in data-centric explainable ML approaches for geospatial data analysis.
- Scripts in R and python to perform geospatial data analysis, available upon request.
This book is an essential resource for graduate students, researchers, and academics working in and studying data science and machine learning, as well as geospatial data science professionals using GIS and remote sensing in environmental fields.
Part I: Introduction. 1. Challenges and Opportunities. Part II: Foundations. 2. An Introduction to Explainable Machine Learning. 3. Approaches to Explainable Machine Learning. 4. Approaches to Explainable Deep Learning. 5. Landslide Susceptibility Modeling Using a Logistic Regression Model. Part III: Techniques and Applications. 6. Urban Land Cover Classification Using Earth Observation (EO) Data and Machine Learning Models. 7. Modeling Forest Canopy Height Using Earth Observation (EO) Data and Machine Learning Models. 8. Modeling Aboveground Biomass Density Using Earth Observation (EO) Data and Machine Learning Models. 9. Explainable Deep Learning for Mapping Building Footprints Using High-Resolution Imagery. 10. Towards Explainable AI and Data-Centric Approaches for Geospatial Data Analysis. 11. Appendix.