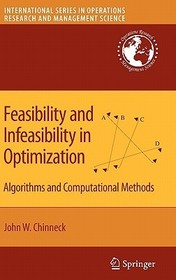
Feasibility and Infeasibility in Optimization:
Algorithms and Computational Methods
Series: International Series in Operations Research & Management Science; 118;
- Publisher's listprice EUR 106.99
-
The price is estimated because at the time of ordering we do not know what conversion rates will apply to HUF / product currency when the book arrives. In case HUF is weaker, the price increases slightly, in case HUF is stronger, the price goes lower slightly.
- Discount 8% (cc. 3 631 Ft off)
- Discounted price 41 753 Ft (39 765 Ft + 5% VAT)
45 385 Ft
Availability
Estimated delivery time: In stock at the publisher, but not at Prospero's office. Delivery time approx. 3-5 weeks.
Not in stock at Prospero.
Why don't you give exact delivery time?
Delivery time is estimated on our previous experiences. We give estimations only, because we order from outside Hungary, and the delivery time mainly depends on how quickly the publisher supplies the book. Faster or slower deliveries both happen, but we do our best to supply as quickly as possible.
Product details:
- Edition number 2008
- Publisher Springer
- Date of Publication 6 December 2007
- Number of Volumes 1 pieces, Book
- ISBN 9780387749310
- Binding Hardback
- No. of pages274 pages
- Size 235x155 mm
- Weight 1310 g
- Language English
- Illustrations XXII, 274 p. Tables, black & white 0
Categories
Short description:
Constrained optimization models are core tools in business, science, government, and the military with applications including airline scheduling, control of petroleum refining operations, investment decisions, and many others. Constrained optimization models have grown immensely in scale and complexity in recent years as inexpensive computing power has become widely available. Models now frequently have many complicated interacting constraints, giving rise to a host of issues related to feasibility and infeasibility. For example, it is sometimes difficult to find any feasible point at all for a large model, or even to accurately determine if one exists, e.g. for nonlinear models. If the model is feasible, how quickly can a solution be found? If the model is infeasible, how can the cause be isolated and diagnosed? Can a repair to restore feasibility be carried out automatically? Researchers have developed numerous algorithms and computational methods in recent years to address such issues, with a number of surprising spin-off applications in fields such as artificial intelligence and computational biology. Over the same time period, related approaches and techniques relating to feasibility and infeasibility of constrained problems have arisen in the constraint programming community.
Feasibility and Infeasibility in Optimization is a timely expository book that summarizes the state of the art in both classical and recent algorithms related to feasibility and infeasibility in optimization, with a focus on practical methods. All model forms are covered, including linear, nonlinear, and mixed-integer programs. Connections to related work in constraint programming are shown. Part I of the book addresses algorithms for seeking feasibility quickly, including new methods for the difficult cases of nonlinear and mixed-integer programs. Part II provides algorithms for analyzing infeasibility by isolating minimal infeasible (or maximum feasible) subsets of constraints, or by finding the best repair for the infeasibility. Infeasibility analysis algorithms have arisen primarily over the last two decades, and the book covers these in depth and detail. Part III describes applications in numerous areas outside of direct infeasibility analysis such as finding decision trees for data classification, analyzing protein folding, radiation treatment planning, automated test assembly, etc.
A main goal of the book is to impart an understanding of the methods so that practitioners can make immediate use of existing algorithms and software, and so that researchers can extend the state of the art and find new applications. The book is of interest to researchers, students, and practitioners across the applied sciences who are working on optimization problems.
More
Long description:
Feasibility and Infeasibility in Optimization is an expository book focused on practical algorithms related to feasibility and infeasibility in optimization. Part I addresses algorithms for seeking feasibility quickly, including recent algorithms for the difficult cases of nonlinear and mixed-integer programs. Part II provides algorithms for analyzing infeasibility by isolating minimal infeasible (or maximum feasible) subsets of constraints, or by finding the best repair for the infeasibility. Part III describes surprising applications in areas such as classification, computational biology, and medicine. Connections to constraint programming are shown. A main goal is to impart an understanding of the methods so that practitioners can make immediate use of existing algorithms and software, and so that researchers can extend the state of the art and find new applications. The book is of interest to researchers, students, and practitioners across the applied sciences who are working on optimization problems.
From the reviews:
"This book is really the first monograph to summarize the growing body of research on the analysis of feasibility and infeasibility of optimization problems. With up to date coverage and very thorough bibliography, it will be of definite interest to researchers working in this area. The book may also be of interest to those readers who are more interested in modeling and applications but want to learn something about techniques for analyzing the feasibility of optimization models." (Brian Borchers, MathDL, March, 2008)
MoreTable of Contents:
Seeking Feasibility.- Preliminaries.- Seeking Feasibility in Linear Programs.- Seeking Feasibility in Mixed-Integer Linear Programs.- A Brief Tour of Constraint Programming.- Seeking Feasibility in Nonlinear Programs.- Analyzing Infeasibility.- Isolating Infeasibility.- Finding the Maximum Feasible Subset of Linear Constraints.- Altering Constraints to Achieve Feasibility.- Applications.- Other Model Analyses.- Data Analysis.- Miscellaneous Applications.- Epilogue.
More