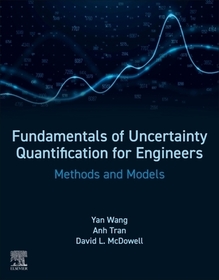
ISBN13: | 9780443136610 |
ISBN10: | 04431366111 |
Binding: | Paperback |
No. of pages: | 600 pages |
Size: | 228x152 mm |
Weight: | 450 g |
Language: | English |
700 |
Fundamentals of Uncertainty Quantification for Engineers
EUR 195.00
Click here to subscribe.
Fundamentals of Uncertainty Quantification for Engineers: Methods and Models provides a comprehensive introduction to uncertainty quantification (UQ) accompanied by a wide variety of applied examples, implementation details, and practical exercises to reinforce the concepts outlined in the book. Sections start with a review of the history of probability theory and recent developments of UQ methods in the domains of applied mathematics and data science. Major concepts of probability axioms, conditional probability, and Bayes’ rule are discussed and examples of probability distributions in parametric data analysis, reliability, risk analysis, and materials informatics are included.
Random processes, sampling methods, and surrogate modeling techniques including multivariate polynomial regression, Gaussian process regression, multi-fidelity surrogate, support-vector machine, and decision tress are also covered. Methods for model selection, calibration, and validation are introduced next, followed by chapters on sensitivity analysis, stochastic expansion methods, Markov models, and non-probabilistic methods. The book concludes with a chapter describing the methods that can be used to predict UQ in systems, such as Monte Carlo, stochastic expansion, upscaling, Langevin dynamics, and inverse problems, with example applications in multiscale modeling, simulations, and materials design.
2. Probability and Statistics in Uncertainty Quantification
3. Random Processes in Uncertainty Quantification
4. Sampling Methods in Uncertainty Quantification
5. Surrogate Modeling in Uncertainty Quantification
6. Model Selection, Calibration, and Validation in Uncertainty Quantification
7. Sensitivity Analysis in Uncertainty Quantification
8. Stochastic Expansion Methods in Uncertainty Quantification
9. Markov Models
10. Non-Probabilistic Methods in Uncertainty Quantification
11. Uncertainty propagation in Uncertainty Quantification