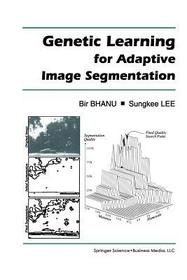
Genetic Learning for Adaptive Image Segmentation
Series: The Springer International Series in Engineering and Computer Science; 287;
- Publisher's listprice EUR 160.49
-
The price is estimated because at the time of ordering we do not know what conversion rates will apply to HUF / product currency when the book arrives. In case HUF is weaker, the price increases slightly, in case HUF is stronger, the price goes lower slightly.
- Discount 8% (cc. 5 446 Ft off)
- Discounted price 62 633 Ft (59 650 Ft + 5% VAT)
68 079 Ft
Availability
Estimated delivery time: In stock at the publisher, but not at Prospero's office. Delivery time approx. 3-5 weeks.
Not in stock at Prospero.
Why don't you give exact delivery time?
Delivery time is estimated on our previous experiences. We give estimations only, because we order from outside Hungary, and the delivery time mainly depends on how quickly the publisher supplies the book. Faster or slower deliveries both happen, but we do our best to supply as quickly as possible.
Product details:
- Edition number Softcover reprint of the original 1st ed. 1994
- Publisher Springer
- Date of Publication 22 December 2012
- Number of Volumes 1 pieces, Book
- ISBN 9781461361985
- Binding Paperback
- No. of pages271 pages
- Size 235x155 mm
- Weight 456 g
- Language English
- Illustrations XIX, 271 p. Illustrations, black & white 0
Categories
Long description:
Image segmentation is generally the first task in any automated image understanding application, such as autonomous vehicle navigation, object recognition, photointerpretation, etc. All subsequent tasks, such as feature extraction, object detection, and object recognition, rely heavily on the quality of segmentation. One of the fundamental weaknesses of current image segmentation algorithms is their inability to adapt the segmentation process as real-world changes are reflected in the image. Only after numerous modifications to an algorithm's control parameters can any current image segmentation technique be used to handle the diversity of images encountered in real-world applications.
Genetic Learning for Adaptive Image Segmentation presents the first closed-loop image segmentation system that incorporates genetic and other algorithms to adapt the segmentation process to changes in image characteristics caused by variable environmental conditions, such as time of day, time of year, weather, etc. Image segmentation performance is evaluated using multiple measures of segmentation quality. These quality measures include global characteristics of the entire image as well as local features of individual object regions in the image.
This adaptive image segmentation system provides continuous adaptation to normal environmental variations, exhibits learning capabilities, and provides robust performance when interacting with a dynamic environment. This research is directed towards adapting the performance of a well known existing segmentation algorithm (Phoenix) across a wide variety of environmental conditions which cause changes in the image characteristics. The book presents a large number of experimental results and compares performance with standard techniques used in computer vision for both consistency and quality of segmentation results. These results demonstrate, (a) the ability to adapt the segmentation performance in both indoor and outdoor color imagery, and (b) that learning from experience can be used to improve the segmentation performance over time.
Springer Book Archives
Table of Contents:
1 Introduction.- 2 Image Segmentation Techniques.- 3 Segmentation as an Optimization Problem.- 4 Baseline Adaptive Image Segmentation Using a Genetic Algorithm.- 5 Basic Experimental Results ? Indoor Imagery.- 6 Basic Experimental Results ? Outdoor Imagery.- 7 Evaluating the Effectiveness of the Baseline Technique ? Further experiments.- 8 Hybrid Search Scheme for Adaptive Image Segmentation.- 9 Simultaneous Optimization of Global and Local Evaluation Measures.- 10 Summary.- References.
More