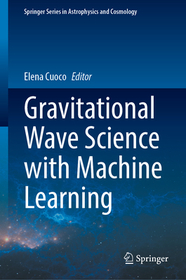
ISBN13: | 9789819617364 |
ISBN10: | 9819617367 |
Binding: | Hardback |
No. of pages: | 289 pages |
Size: | 235x155 mm |
Language: | English |
Illustrations: | 4 Illustrations, black & white; 104 Illustrations, color |
700 |
Gravitational Wave Science with Machine Learning
EUR 149.79
Click here to subscribe.
This book highlights the state of the art of machine learning applied to the science of gravitational waves. The main topics of the book range from the search for astrophysical gravitational wave signals to noise suppression techniques and control systems using machine learning-based algorithms. During the four years of work in the COST Action CA17137-A network for Gravitational Waves, Geophysics and Machine Learning (G2net), the collaboration produced several original publications as well as tutorials and lectures in the training schools we organized. The book encapsulates the immense amount of finding and achievements.
It is a timely reference for young researchers approaching the analysis of data from gravitational wave experiments, with alternative approaches based on the use of artificial intelligence techniques.
This book highlights the state of the art of machine learning applied to the science of gravitational waves. The main topics of the book range from the search for astrophysical gravitational wave signals to noise suppression techniques and control systems using machine learning-based algorithms. During the four years of work in the COST Action CA17137-A network for Gravitational Waves, Geophysics and Machine Learning (G2net), the collaboration produced several original publications as well as tutorials and lectures in the training schools we organized. The book encapsulates the immense amount of finding and achievements.
It is a timely reference for young researchers approaching the analysis of data from gravitational wave experiments, with alternative approaches based on the use of artificial intelligence techniques.
1. Neural network time-series classifiers for gravitational-wave searches in single-detector periods.- 2. A simple self similarity-based unsupervised noise monitor for gravitational-wave detectors.- 3 Simulation of transient noise bursts in gravitational wave interferometers.- 4. Efficient ML Algorithms for Detecting Glitches and Data Patterns in LIGO Time Series.- 5. Denoising gravitational-wave signals from binary black holes with dilated convolutional autoencoder.