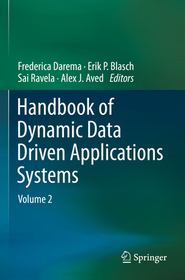
Handbook of Dynamic Data Driven Applications Systems
Volume 2
- Publisher's listprice EUR 192.59
-
The price is estimated because at the time of ordering we do not know what conversion rates will apply to HUF / product currency when the book arrives. In case HUF is weaker, the price increases slightly, in case HUF is stronger, the price goes lower slightly.
- Discount 8% (cc. 6 536 Ft off)
- Discounted price 75 161 Ft (71 582 Ft + 5% VAT)
81 696 Ft
Availability
Estimated delivery time: In stock at the publisher, but not at Prospero's office. Delivery time approx. 3-5 weeks.
Not in stock at Prospero.
Why don't you give exact delivery time?
Delivery time is estimated on our previous experiences. We give estimations only, because we order from outside Hungary, and the delivery time mainly depends on how quickly the publisher supplies the book. Faster or slower deliveries both happen, but we do our best to supply as quickly as possible.
Product details:
- Edition number 2023
- Publisher Springer
- Date of Publication 15 September 2024
- Number of Volumes 1 pieces, Book
- ISBN 9783031279881
- Binding Paperback
- No. of pages956 pages
- Size 235x155 mm
- Language English
- Illustrations 35 Illustrations, black & white; 281 Illustrations, color 646
Categories
Short description:
This Second Volume in the series Handbook of Dynamic Data Driven Applications Systems (DDDAS) expands the scope of the methods and the application areas presented in the first Volume and aims to provide additional and extended content of the increasing set of science and engineering advances for new capabilities enabled through DDDAS. The methods and examples of breakthroughs presented in the book series capture the DDDAS paradigm and its scientific and technological impact and benefits. The DDDAS paradigm and the ensuing DDDAS-based frameworks for systems? analysis and design have been shown to engender new and advanced capabilities for understanding, analysis, and management of engineered, natural, and societal systems (?applications systems?), and for the commensurate wide set of scientific and engineering fields and applications, as well as foundational areas. The DDDAS book series aims to be a reference source ofmany of the important research and development efforts conducted under the rubric of DDDAS through the examples and case studies presented, either within their own field or other fields of study.
DDDAS has proven to be a transformative technology as data has become the third part of the triad, with theory and computation as the other two parts. Initially DDDAS was part of control theory but as data have become ubiquitous there has been a paradigm shift, initiated by DDDAS, from simulation to effectively using data for prediction.
John Cherniavsky, retired, as Division Director, NSF - US National Science Foundation
The DDDAS paradigm integrates and enhances the deepest, most well-grounded foundations and tools - both from expert based methods and from learning-based methods, building well beyond more popular and limited forms of AI. This book provides a unique breadth and depth of scope across many science and technology fields, showing how DDDAS is an overarching concept that connects and unifies the wide diversity across these fields.
Paul Werbos, retired, as Program Director, NSF - US National Science Foundation
Long description:
This Second Volume in the series Handbook of Dynamic Data Driven Applications Systems (DDDAS) expands the scope of the methods and the application areas presented in the first Volume and aims to provide additional and extended content of the increasing set of science and engineering advances for new capabilities enabled through DDDAS. The methods and examples of breakthroughs presented in the book series capture the DDDAS paradigm and its scientific and technological impact and benefits. The DDDAS paradigm and the ensuing DDDAS-based frameworks for systems? analysis and design have been shown to engender new and advanced capabilities for understanding, analysis, and management of engineered, natural, and societal systems (?applications systems?), and for the commensurate wide set of scientific and engineering fields and applications, as well as foundational areas. The DDDAS book series aims to be a reference source of many of the important research and development efforts conducted under the rubric of DDDAS, and to also inspire the broader communities of researchers and developers about the potential in their respective areas of interest, of the application and the exploitation of the DDDAS paradigm and the ensuing frameworks, through the examples and case studies presented, either within their own field or other fields of study.
As in the first volume, the chapters in this book reflect research work conducted over the years starting in the 1990?s to the present. Here, the theory and application content are considered for:- Foundational Methods
- Materials Systems
- Structural Systems
- Energy Systems
- Environmental Systems: Domain Assessment & Adverse Conditions/Wildfires
- Surveillance Systems
- Space Awareness Systems
- Healthcare Systems
- Decision Support Systems
- Cyber Security Systems
- Design of Computer Systems
More
Table of Contents:
The Dynamic Data Driven Applications Systems (DDDAS) Paradigm and Emerging Directions.- Dynamic Data Driven Applications Systems and Information-Inference Couplings.- Polynomial Chaos Expansion Based Nonlinear Filtering for Dynamic State Estimation.- Measure-Invariant Symbolic Systems for Pattern Recognition and Anomaly Detection.- Equation?Free Computations as DDDAS Protocols for Bifurcation Studies: A Granular Chain Example.- A Stochastic Dynamic Data-Driven Framework for Real-time Prediction of Materials Damage in Composites.- Dynamic Data-Driven Monitoring of Nanoparticle Self Assembly Processes.- From Data to Decisions: A Real-Time Measurement -Inversion-Prediction-Steering Framework for Hazardous Events and Structural Health Monitoring.- Bayesian Computational Sensor Networks: Small-Scale Structural Health Monitoring.- A Dynamic Data Driven Sensor Tasking with Application of Aerospace Systems.- Dynamic Data-Driven Application Systems for Reservoir Simulation-Based Optimization: Lessons Learned and Future Trends.- DDDAS within the Oil and Gas Industry.- A Simulation-Based Online Dynamic Data-Driven Framework for Large-Scale Wind-turbine Farm Systems Operation.- Towards Dynamic Data Driven Systems for Rapid Adaptive Interdisciplinary Ocean Forecasting.- Towards Cyber-Eco Systems: Networked Sensing, Inference and Control for Ecological and Agricultural Systems.- An Energy-Aware Airborne Dynamic Data-Driven Application System for Persistent Sampling and Surveillance.- Using Dynamic Data Driven Cyberinfrastructure for Next Generation Wildland Fire Intelligence.- Autonomous Monitoring of Wildfires with Vision-Equipped UAS and Temperature Sensors via Evidential Reasoning.- Airborne Fire Detection and Modeling using Unmanned Aerial Vehicles Imagery: Datasets and Approaches.- DDDAS-based Remote Sensing.- Advances in Domain Adaptation for Aerial Imagery.- Retrospective Cost Parameter Estimation with Application to Space Weather Modeling.- A Dynamic Data Driven Approach to Space Situational Awareness.- Data driven cancer research with digital microscopy and Pathomics.- Robust Data Driven Region of Interest Segmentation for Breast Thermography.- Adaptive Data Stream Mining (DSM) Systems.- Deception Detection in Videos using Robust Facial Features with Attention Feedback.- Manufacturing the Future via Dynamic Data Driven Applications Systems (DDDAS).- DDDAS in the Social Sciences.- Anomaly-Detection Defense against Test-Time Evasion Attacks on Robust DNNs.- Dynamic Data-Driven Approach for Cyber Resilient and Secure Critical Energy Systems.- Dynamic Network-centric Multi-cloud Platform for Real-Time and Data-Intensive Science Workflows.- INDICES: Applying DDDAS Principles for Performance Interference?aware Cloud?to?Fog Application Migration.- Adaptive Routing for Hybrid Photonic-Plasmonic (HyPPI) Interconnection Network for Manycore Processors using DDDAS on the Chip.
More