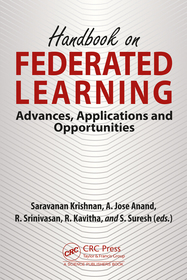
Handbook on Federated Learning
Advances, Applications and Opportunities
- Publisher's listprice GBP 135.00
-
The price is estimated because at the time of ordering we do not know what conversion rates will apply to HUF / product currency when the book arrives. In case HUF is weaker, the price increases slightly, in case HUF is stronger, the price goes lower slightly.
- Discount 10% (cc. 6 832 Ft off)
- Discounted price 61 491 Ft (58 563 Ft + 5% VAT)
68 323 Ft
Availability
Estimated delivery time: In stock at the publisher, but not at Prospero's office. Delivery time approx. 3-5 weeks.
Not in stock at Prospero.
Why don't you give exact delivery time?
Delivery time is estimated on our previous experiences. We give estimations only, because we order from outside Hungary, and the delivery time mainly depends on how quickly the publisher supplies the book. Faster or slower deliveries both happen, but we do our best to supply as quickly as possible.
Product details:
- Edition number 1
- Publisher CRC Press
- Date of Publication 15 December 2023
- ISBN 9781032471624
- Binding Hardback
- No. of pages362 pages
- Size 234x156 mm
- Weight 453 g
- Language English
- Illustrations 77 Illustrations, black & white; 14 Illustrations, color; 17 Tables, black & white 579
Categories
Short description:
Federated learning is a Distributed Machine Learning model that has been used in many applications today. Most edge devices can execute models with local dataset since their computation power is unutilized.
MoreLong description:
Mobile, wearable, and self-driving telephones are just a few examples of modern distributed networks that generate enormous amount of information every day. Due to the growing computing capacity of these devices as well as concerns over the transfer of private information, it has become important to process the part of the data locally by moving the learning methods and computing to the border of devices. Federated learning has developed as a model of education in these situations. Federated learning (FL) is an expert form of decentralized machine learning (ML). It is essential in areas like privacy, large-scale machine education and distribution. It is also based on the current stage of ICT and new hardware technology and is the next generation of artificial intelligence (AI). In FL, central ML model is built with all the data available in a centralised environment in the traditional machine learning. It works without problems when the predictions can be served by a central server. Users require fast responses in mobile computing, but the model processing happens at the sight of the server, thus taking too long. The model can be placed in the end-user device, but continuous learning is a challenge to overcome, as models are programmed in a complete dataset and the end-user device lacks access to the entire data package. Another challenge with traditional machine learning is that user data is aggregated at a central location where it violates local privacy policies laws and make the data more vulnerable to data violation. This book provides a comprehensive approach in federated learning for various aspects.
MoreTable of Contents:
Introduction to Federated Learning: Methods, and Classifications. Go Local, Go Global and Go Fusion - How to pick data from various contexts. Federated Learning Architectures, Opportunities, and Applications. Secure and Private Federated Learning through Encrypted Parameter Aggregation. Navigating Privacy Concerns in Federated Learning: A GDPR-Focused Analysis. A Federated Learning Approach for Resource-Constrained IoT Security Monitoring. Efficient Federated Learning Techniques for Data Loss Prevention in Cloud Environment. Maximizing Fog Computing Efficiency with Federated Multi-Agent Deep Reinforcement Learning. Future of Medical Research with a data-driven Federated Learning Approach. Collaborative Federated Learning in Healthcare Systems. Federated Learning for Efficient Cardiac Disease Prediction based on Hyper Spectral Feature Selection using Deep Spectral Convolution Neural Network. A Federated Learning based Alzheimer?s Disease Prediction. Detecting Device Sensors of Luxury Hotel Using Blockchain Based Federated Learning to Increase Customer Satisfaction. Navigating the Complexity of Macro-Tasks: Federated Learning as a Catalyst for Effective Crowd Coordination. Stock Market Prediction via Twitter Sentiment Analysis using BERT: A Federated Learning Approach.
More