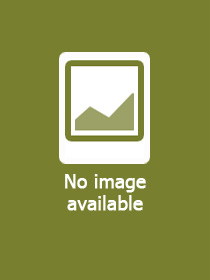
Product details:
ISBN13: | 9780443265105 |
ISBN10: | 04432651011 |
Binding: | Paperback |
No. of pages: | 442 pages |
Size: | 234x190 mm |
Weight: | 450 g |
Language: | English |
699 |
Category:
Implementation and Interpretation of Machine and Deep Learning to Applied Subsurface Geological Problems
Prediction Models Exploiting Well-Log Information
Publisher: Elsevier
Date of Publication: 24 February 2025
Normal price:
Publisher's listprice:
EUR 153.99
EUR 153.99
Your price:
60 245 (57 376 HUF + 5% VAT )
discount is: 10% (approx 6 694 HUF off)
The discount is only available for 'Alert of Favourite Topics' newsletter recipients.
Click here to subscribe.
Click here to subscribe.
Availability:
Not yet published.
Long description:
Implementation and Interpretation of Machine and Deep Learning to Applied Subsurface Geological Problems: Prediction Models Exploiting Well-Log Information explores machine and deep learning models for subsurface geological prediction problems commonly encountered in applied resource evaluation and reservoir characterization tasks. The book provides insights into how the performance of ML/DL models can be optimized-and sparse datasets of input variables enhanced and/or rescaled-to improve prediction performances. A variety of topics are covered, including regression models to estimate total organic carbon from well-log data, predicting brittleness indexes in tight formation sequences, trapping mechanisms in potential sub-surface carbon storage reservoirs, and more.
Each chapter includes its own introduction, summary, and nomenclature sections, along with one or more case studies focused on prediction model implementation related to its topic.
Each chapter includes its own introduction, summary, and nomenclature sections, along with one or more case studies focused on prediction model implementation related to its topic.
Table of Contents:
1. Regression models to estimate total organic carbon (TOC) from well-log data
2. Predicting brittleness indexes in tight formation sequences
3. Classifying lithofacies in clastic, carbonate, and mixed reservoir sequences
4. Permeability and water saturation distributions in complex reservoirs
5. Trapping mechanisms in potential sub-surface carbon storage reservoirs
6. The accurate picking of formation tops in field development wells
7. Assessing formation loss of circulation risks with mud-log datasets
8. Delineating fracture densities and apertures using well-log image data
9. Determining reservoir microfacies using photomicrograph and computed tomography image data
10. Characterizing coal-bed methane reservoirs with well-log datasets
2. Predicting brittleness indexes in tight formation sequences
3. Classifying lithofacies in clastic, carbonate, and mixed reservoir sequences
4. Permeability and water saturation distributions in complex reservoirs
5. Trapping mechanisms in potential sub-surface carbon storage reservoirs
6. The accurate picking of formation tops in field development wells
7. Assessing formation loss of circulation risks with mud-log datasets
8. Delineating fracture densities and apertures using well-log image data
9. Determining reservoir microfacies using photomicrograph and computed tomography image data
10. Characterizing coal-bed methane reservoirs with well-log datasets