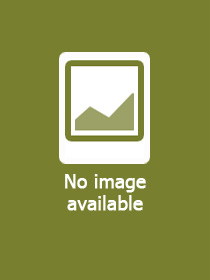
Product details:
ISBN13: | 9783030916978 |
ISBN10: | 3030916979 |
Binding: | Paperback |
No. of pages: | 685 pages |
Size: | 235x155 mm |
Weight: | 1062 g |
Language: | English |
Illustrations: | 7 Illustrations, black & white; 2 Illustrations, color |
518 |
Category:
Large Sample Techniques for Statistics
Series:
Springer Texts in Statistics;
Edition number: Second Edition 2022
Publisher: Springer
Date of Publication: 6 April 2023
Number of Volumes: 1 pieces, Book
Normal price:
Publisher's listprice:
EUR 74.89
EUR 74.89
Your price:
29 950 (28 524 HUF + 5% VAT )
discount is: 8% (approx 2 604 HUF off)
The discount is only available for 'Alert of Favourite Topics' newsletter recipients.
Click here to subscribe.
Click here to subscribe.
Availability:
Estimated delivery time: In stock at the publisher, but not at Prospero's office. Delivery time approx. 3-5 weeks.
Not in stock at Prospero.
Can't you provide more accurate information?
Not in stock at Prospero.
Short description:
This book offers a comprehensive guide to large sample techniques in statistics. With a focus on developing analytical skills and understanding motivation, Large Sample Techniques for Statistics begins with fundamental techniques, and connects theory and applications in engaging ways.
This book offers a comprehensive guide to large sample techniques in statistics. With a focus on developing analytical skills and understanding motivation, Large Sample Techniques for Statistics begins with fundamental techniques, and connects theory and applications in engaging ways.
The first five chapters review some of the basic techniques, such as the fundamental epsilon-delta arguments, Taylor expansion, different types of convergence, and inequalities. The next five chapters discuss limit theorems in specific situations of observational data. Each of the first ten chapters contains at least one section of case study. The last six chapters are devoted to special areas of applications. This new edition introduces a final chapter dedicated to random matrix theory, as well as expanded treatment of inequalities and mixed effects models.
The book's case studies and applications-oriented chapters demonstrate how to usemethods developed from large sample theory in real world situations. The book is supplemented by a large number of exercises, giving readers opportunity to practice what they have learned. Appendices provide context for matrix algebra and mathematical statistics. The Second Edition seeks to address new challenges in data science.
This text is intended for a wide audience, ranging from senior undergraduate students to researchers with doctorates. A first course in mathematical statistics and a course in calculus are prerequisites..
Long description:
This book offers a comprehensive guide to large sample techniques in statistics. With a focus on developing analytical skills and understanding motivation, Large Sample Techniques for Statistics begins with fundamental techniques, and connects theory and applications in engaging ways.
The first five chapters review some of the basic techniques, such as the fundamental epsilon-delta arguments, Taylor expansion, different types of convergence, and inequalities. The next five chapters discuss limit theorems in specific situations of observational data. Each of the first ten chapters contains at least one section of case study. The last six chapters are devoted to special areas of applications. This new edition introduces a final chapter dedicated to random matrix theory, as well as expanded treatment of inequalities and mixed effects models.
The book's case studies and applications-oriented chapters demonstrate how to use methods developed from large sample theory in real world situations. The book is supplemented by a large number of exercises, giving readers opportunity to practice what they have learned. Appendices provide context for matrix algebra and mathematical statistics. The Second Edition seeks to address new challenges in data science.
This text is intended for a wide audience, ranging from senior undergraduate students to researchers with doctorates. A first course in mathematical statistics and a course in calculus are prerequisites..
Table of Contents:
Chapter 1. The -? Arguments.- Chapter 2. Modes of Convergence.- Chapter 3. Big O, Small o, and the Unspecified c.- Chapter 4. Asymptotic Expansions.- Chapter 5. Inequalities.- Chapter 6. Sums of Independent Random Variables.- Chapter 7. Empirical Processes.- Chapter 8. Martingales.- Chapter 9. Time and Spatial Series.- Chapter 10. Stochastic Processes.- Chapter 11. Nonparametric Statistics.- Chapter 12. Mixed Effects Models.- Chapter 13. Small-Area Estimation.- Chapter 14. Jackknife and Bootstrap.- Chapter 15. Markov-Chain Monte Carlo.- Chapter 16. Random Matrix Theory.