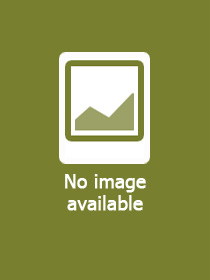
Product details:
ISBN13: | 9789811290688 |
ISBN10: | 9811290687 |
Binding: | Hardback |
No. of pages: | 288 pages |
Language: | English |
700 |
Category:
Latent Structure And Causality: Inference From Data
Publisher: World Scientific
Date of Publication: 21 April 2025
Normal price:
Publisher's listprice:
GBP 80.00
GBP 80.00
Your price:
37 249 (35 475 HUF + 5% VAT )
discount is: 8% (approx 3 239 HUF off)
The discount is only available for 'Alert of Favourite Topics' newsletter recipients.
Click here to subscribe.
Click here to subscribe.
Availability:
Not yet published.
Long description:
Inferring latent structure and causality is crucial for understanding underlying patterns and relationships hidden in the data. This book covers selected models for latent structures and causal networks and inference methods for these models.After an introduction to the EM algorithm on incomplete data, the book provides a detailed coverage of a few widely used latent structure models, including mixture models, hidden Markov models, and stochastic block models. EM and variation EM algorithms are developed for parameter estimation under these models, with comparison to their Bayesian inference counterparts. We make further extensions of these models to related problems, such as clustering, motif discovery, Kalman filtering, and exchangeable random graphs. Conditional independence structures are utilized to infer the latent structures in the above models, which can be represented graphically. This notion generalizes naturally to the second part on graphical models that use graph separation to encode conditional independence. We cover a variety of graphical models, including undirected graphs, directed acyclic graphs (DAGs), chain graphs, and acyclic directed mixed graphs (ADMGs), and various Markov properties for these models. Recent methods that learn the structure of a graphical model from data are reviewed and discussed. In particular, DAGs and Bayesian networks are an important class of mathematical models for causality. After an introduction to causal inference with DAGs and structural equation models, we provide a detailed review of recent research on causal discovery via structure learning of graphs. Finally, we briefly introduce the causal bandit problem with sequential intervention.