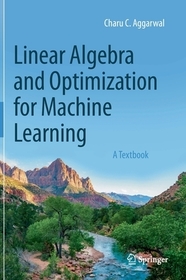
Linear Algebra and Optimization for Machine Learning
A Textbook
- Publisher's listprice EUR 64.19
-
The price is estimated because at the time of ordering we do not know what conversion rates will apply to HUF / product currency when the book arrives. In case HUF is weaker, the price increases slightly, in case HUF is stronger, the price goes lower slightly.
- Discount 8% (cc. 2 178 Ft off)
- Discounted price 25 050 Ft (23 857 Ft + 5% VAT)
27 229 Ft
Availability
Estimated delivery time: In stock at the publisher, but not at Prospero's office. Delivery time approx. 3-5 weeks.
Not in stock at Prospero.
Why don't you give exact delivery time?
Delivery time is estimated on our previous experiences. We give estimations only, because we order from outside Hungary, and the delivery time mainly depends on how quickly the publisher supplies the book. Faster or slower deliveries both happen, but we do our best to supply as quickly as possible.
Product details:
- Edition number 1st ed. 2020
- Publisher Springer
- Date of Publication 13 May 2020
- Number of Volumes 1 pieces, Book
- ISBN 9783030403430
- Binding Hardback
- No. of pages495 pages
- Size 254x178 mm
- Weight 1184 g
- Language English
- Illustrations 67 Illustrations, black & white; 26 Illustrations, color 120
Categories
Short description:
This textbook introduces linear algebra and optimization in the context of machine learning. Examples and exercises are provided throughout the book. A solution manual for the exercises at the end of each chapter is available to teaching instructors. This textbook targets graduate level students and professors in computer science, mathematics and data science. Advanced undergraduate students can also use this textbook. The chapters for this textbook are organized as follows:
1. Linear algebra and its applications: The chapters focus on the basics of linear algebra together with their common applications to singular value decomposition, matrix factorization, similarity matrices (kernel methods), and graph analysis. Numerous machine learning applications have been used as examples, such as spectral clustering, kernel-based classification, and outlier detection. The tight integration of linear algebra methods with examples from machine learning differentiates this book fromgeneric volumes on linear algebra. The focus is clearly on the most relevant aspects of linear algebra for machine learning and to teach readers how to apply these concepts.
2. Optimization and its applications: Much of machine learning is posed as an optimization problem in which we try to maximize the accuracy of regression and classification models. The ?parent problem? of optimization-centric machine learning is least-squares regression. Interestingly, this problem arises in both linear algebra and optimization, and is one of the key connecting problems of the two fields. Least-squares regression is also the starting point for support vector machines, logistic regression, and recommender systems. Furthermore, the methods for dimensionality reduction and matrix factorization also require the development of optimization methods. A general view of optimization in computational graphs is discussed together with its applications to back propagation in neural networks.
A frequent challenge faced by beginners in machine learning is the extensive background required in linear algebra and optimization. One problem is that the existing linear algebra and optimization courses are not specific to machine learning; therefore, one would typically have to complete more course material than is necessary to pick up machine learning. Furthermore, certain types of ideas and tricks from optimization and linear algebra recur more frequently in machine learning than other application-centric settings. Therefore, there is significant value in developing a view of linear algebra and optimization that is better suited to the specific perspective of machine learning.
MoreLong description:
This textbook introduces linear algebra and optimization in the context of machine learning. Examples and exercises are provided throughout the book. A solution manual for the exercises at the end of each chapter is available to teaching instructors. This textbook targets graduate level students and professors in computer science, mathematics and data science. Advanced undergraduate students can also use this textbook. The chapters for this textbook are organized as follows:
1. Linear algebra and its applications: The chapters focus on the basics of linear algebra together with their common applications to singular value decomposition, matrix factorization, similarity matrices (kernel methods), and graph analysis. Numerous machine learning applications have been used as examples, such as spectral clustering, kernel-based classification, and outlier detection. The tight integration of linear algebra methods with examples from machine learning differentiates this book from generic volumes on linear algebra. The focus is clearly on the most relevant aspects of linear algebra for machine learning and to teach readers how to apply these concepts.
2. Optimization and its applications: Much of machine learning is posed as an optimization problem in which we try to maximize the accuracy of regression and classification models. The ?parent problem? of optimization-centric machine learning is least-squares regression. Interestingly, this problem arises in both linear algebra and optimization, and is one of the key connecting problems of the two fields. Least-squares regression is also the starting point for support vector machines, logistic regression, and recommender systems. Furthermore, the methods for dimensionality reduction and matrix factorization also require the development of optimization methods. A general view of optimization in computational graphs is discussed together with its applications to back propagation in neural networks.
A frequent challenge faced by beginners in machine learning is the extensive background required in linear algebra and optimization. One problem is that the existing linear algebra and optimization courses are not specific to machine learning; therefore, one would typically have to complete more course material than is necessary to pick up machine learning. Furthermore, certain types of ideas and tricks from optimization and linear algebra recur more frequently in machine learning than other application-centric settings. Therefore, there is significant value in developing a view of linear algebra and optimization that is better suited to the specific perspective of machine learning.
?Based on the topics covered and the excellent presentation, I would recommend Aggarwal's book over these other books for an advanced undergraduate or beginning graduate course on mathematics for data science.? (Brian Borchers, MAA Reviews, March 28, 2021)
?This book should be of interest to graduate students in engineering, applied mathematics, and other fields requiring an understanding of the mathematical underpinnings of machine learning.? (IEEE Control Systems Magazine, Vol. 40 (6), December, 2020)
MoreTable of Contents:
Preface.- 1 Linear Algebra and Optimization: An Introduction.- 2 Linear Transformations and Linear Systems.- 3 Eigenvectors and Diagonalizable Matrices.- 4 Optimization Basics: A Machine Learning View.- 5 Advanced Optimization Solutions.- 6 Constrained Optimization and Duality.- 7 Singular Value Decomposition.- 8 Matrix Factorization.- 9 The Linear Algebra of Similarity.- 10 The Linear Algebra of Graphs.- 11 Optimization in Computational Graphs.- Index.
More