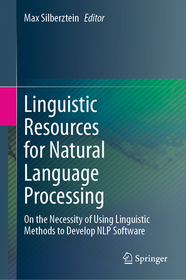
ISBN13: | 9783031438103 |
ISBN10: | 3031438108 |
Binding: | Hardback |
No. of pages: | 217 pages |
Size: | 235x155 mm |
Language: | English |
Illustrations: | 17 Illustrations, black & white; 101 Illustrations, color |
714 |
Linguistic Resources for Natural Language Processing
EUR 163.49
Click here to subscribe.
Not in stock at Prospero.
Empirical ? data-driven, neural network-based, probabilistic, and statistical ? methods seem to be the modern trend. Recently, OpenAI?s ChatGPT, Google?s Bard and Microsoft?s Sydney chatbots have been garnering a lot of attention for their detailed answers across many knowledge domains. In consequence, most AI researchers are no longer interested in trying to understand what common intelligence is or how intelligent agents construct scenarios to solve various problems. Instead, they now develop systems that extract solutions from massive databases used as cheat sheets. In the same manner, Natural Language Processing (NLP) software that uses training corpora associated with empirical methods are trendy, as most researchers in NLP today use large training corpora, always to the detriment of the development of formalized dictionaries and grammars.
Not questioning the intrinsic value of many software applications based on empirical methods, this volume aims at rehabilitating the linguistic approach to NLP. In an introduction, the editor uncovers several limitations and flaws of using training corpora to develop NLP applications, even the simplest ones, such as automatic taggers.
The first part of the volume is dedicated to showing how carefully handcrafted linguistic resources could be successfully used to enhance current NLP software applications. The second part presents two representative cases where data-driven approaches cannot be implemented simply because there is not enough data available for low-resource languages. The third part addresses the problem of how to treat multiword units in NLP software, which is arguably the weakest point of NLP applications today but has a simple and elegant linguistic solution.It is the editor's belief that readers interested in Natural Language Processing will appreciate the importance of this volume, both for its questioning of the training corpus-based approaches and for the intrinsic value of the linguistic formalization and the underlying methodology presented.
Empirical ? data-driven, neural network-based, probabilistic, and statistical ? methods seem to be the modern trend. Recently, OpenAI?s ChatGPT, Google?s Bard and Microsoft?s Sydney chatbots have been garnering a lot of attention for their detailed answers across many knowledge domains. In consequence, most AI researchers are no longer interested in trying to understand what common intelligence is or how intelligent agents construct scenarios to solve various problems. Instead, they now develop systems that extract solutions from massive databases used as cheat sheets. In the same manner, Natural Language Processing (NLP) software that uses training corpora associated with empirical methods are trendy, as most researchers in NLP today use large training corpora, always to the detriment of the development of formalized dictionaries and grammars.
Not questioning the intrinsic value of many software applications based on empirical methods, this volume aims at rehabilitating the linguistic approach to NLP. In an introduction, the editor uncovers several limitations and flaws of using training corpora to develop NLP applications, even the simplest ones, such as automatic taggers.
The first part of the volume is dedicated to showing how carefully handcrafted linguistic resources could be successfully used to enhance current NLP software applications. The second part presents two representative cases where data-driven approaches cannot be implemented simply because there is not enough data available for low-resource languages. The third part addresses the problem of how to treat multiword units in NLP software, which is arguably the weakest point of NLP applications today but has a simple and elegant linguistic solution.It is the editor's belief that readers interested in Natural Language Processing will appreciate the importance of this volume, both for its questioning of the training corpus-based approaches and for the intrinsic value of the linguistic formalization and the underlying methodology presented.
In honor of Peter.- Foreword. - Preface.- About this book. Part 1. Introduction.- 1. The Limitations of Corpus-based Methods in NLP.- Part 2.- 2. Developing Linguistic-based NLP Software.- 3. Linguistic Resources for the Automatic Generation of Texts in Natural Language.- 4. Towards a More Efficient Arabic-French Translation.- 5. Linguistic Resources and Methods and Algorithms for Belarusian Natural Language Processing.- Part 3.- Linguistic Resources for Low-resource Languages.- 6. A New Set of Linguistic Resources for Ukrainian.- 7. Formalization of the Quechua Morphology.- 8. The Challenging Task of Translating the Language of Tango.- 9. A Polylectal Linguistic Resource for Rromani.- Part 4. Processing Multiword Units: The Linguistic Approach.- 10. Using Linguistic Criteria to Define Multiword Units.- 11. A Linguistic Approach to English Phrasal Verbs.- 12. Analysis of Indonesian Multiword Expressions: Linguistic vs Data-driven Approach.