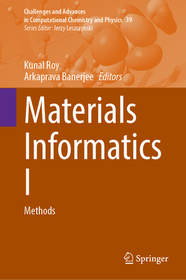
ISBN13: | 9783031787355 |
ISBN10: | 3031787358 |
Binding: | Hardback |
No. of pages: | 338 pages |
Size: | 235x155 mm |
Language: | English |
Illustrations: | 9 Illustrations, black & white; 55 Illustrations, color |
700 |
Materials Informatics I
EUR 249.99
Click here to subscribe.
This contributed volume explores the integration of machine learning and cheminformatics within materials science, focusing on predictive modeling techniques. It begins with foundational concepts in materials informatics and cheminformatics, emphasizing quantitative structure-property relationships (QSPR). The volume then presents various methods and tools, including advanced QSPR models, quantitative read-across structure-property relationship (q-RASPR) models, optimization strategies with minimal data, and in silico studies using different descriptors. Additionally, it explores machine learning algorithms and their applications in materials science, alongside innovative modeling approaches for quantum-theoretic properties. Overall, the book serves as a comprehensive resource for understanding and applying machine learning in the study and development of advanced materials and is a useful tool for students, researchers and professionals working in these areas.
This contributed volume explores the integration of machine learning and cheminformatics within materials science, focusing on predictive modeling techniques. It begins with foundational concepts in materials informatics and cheminformatics, emphasizing quantitative structure-property relationships (QSPR). The volume then presents various methods and tools, including advanced QSPR models, quantitative read-across structure-property relationship (q-RASPR) models, optimization strategies with minimal data, and in silico studies using different descriptors. Additionally, it explores machine learning algorithms and their applications in materials science, alongside innovative modeling approaches for quantum-theoretic properties. Overall, the book serves as a comprehensive resource for understanding and applying machine learning in the study and development of advanced materials and is a useful tool for students, researchers and professionals working in these areas.
Part 1. Introduction.- Introduction to Materials Informatics.- Introduction to Cheminformatics for Predictive Modeling.- Introduction to machine learning for predictive modeling of organic materials.- Quantitative Structure-Property Relationships (QSPR) for Materials Science.- Part 2. Methods and Tools.- Quantitative Structure-Property Relationships (QSPR) and Machine Learning (ML) Models for Materials Science.- Optimising Materials Properties with Minimal Data: Lessons from Vanadium Catalyst Modelling.- In silico QSPR studies based on CDFT and IT descriptors.- Applications of quantitative read-across structure-property relationship (q-RASPR) modeling in the field of materials science.- Machine Learning algorithms for applications in Materials Science I.- Machine Learning algorithms for applications in Materials Science II.- Structure-property modeling of quantum-theoretic properties of benzenoid hydrocarbons by means of connection-related graphical descriptors.- Machine learning tools and Web services for Materials Science modelling.