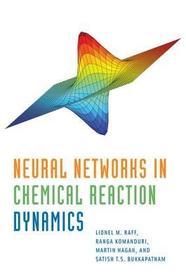
Neural Networks in Chemical Reaction Dynamics
- Publisher's listprice GBP 105.00
-
The price is estimated because at the time of ordering we do not know what conversion rates will apply to HUF / product currency when the book arrives. In case HUF is weaker, the price increases slightly, in case HUF is stronger, the price goes lower slightly.
- Discount 10% (cc. 5 314 Ft off)
- Discounted price 47 826 Ft (45 549 Ft + 5% VAT)
53 140 Ft
Availability
printed on demand
Why don't you give exact delivery time?
Delivery time is estimated on our previous experiences. We give estimations only, because we order from outside Hungary, and the delivery time mainly depends on how quickly the publisher supplies the book. Faster or slower deliveries both happen, but we do our best to supply as quickly as possible.
Product details:
- Publisher OUP USA
- Date of Publication 16 February 2012
- ISBN 9780199765652
- Binding Hardback
- No. of pages312 pages
- Size 160x239x25 mm
- Weight 567 g
- Language English
- Illustrations 109 b&w, 4 color 0
Categories
Short description:
This monograph presents recent advances in neural network (NN) approaches and applications to chemical reaction dynamics.
MoreLong description:
This monograph presents recent advances in neural network (NN) approaches and applications to chemical reaction dynamics. Topics covered include: (i) the development of ab initio potential-energy surfaces (PES) for complex multichannel systems using modified novelty sampling and feedforward NNs; (ii) methods for sampling the configuration space of critical importance, such as trajectory and novelty sampling methods and gradient fitting methods; (iii) parametrization of interatomic potential functions using a genetic algorithm accelerated with a NN; (iv) parametrization of analytic interatomic potential functions using NNs; (v) self-starting methods for obtaining analytic PES from ab inito electronic structure calculations using direct dynamics; (vi) development of a novel method, namely, combined function derivative approximation (CFDA) for simultaneous fitting of a PES and its corresponding force fields using feedforward neural networks; (vii) development of generalized PES using many-body expansions, NNs, and moiety energy approximations; (viii) NN methods for data analysis, reaction probabilities, and statistical error reduction in chemical reaction dynamics; (ix) accurate prediction of higher-level electronic structure energies (e.g. MP4 or higher) for large databases using NNs, lower-level (Hartree-Fock) energies, and small subsets of the higher-energy database; and finally (x) illustrative examples of NN applications to chemical reaction dynamics of increasing complexity starting from simple near equilibrium structures (vibrational state studies) to more complex non-adiabatic reactions.
The monograph is prepared by an interdisciplinary group of researchers working as a team for nearly two decades at Oklahoma State University, Stillwater, OK with expertise in gas phase reaction dynamics; neural networks; various aspects of MD and Monte Carlo (MC) simulations of nanometric cutting, tribology, and material properties at nanoscale; scaling laws from atomistic to continuum; and neural networks applications to chemical reaction dynamics. It is anticipated that this emerging field of NN in chemical reaction dynamics will play an increasingly important role in MD, MC, and quantum mechanical studies in the years to come.
Table of Contents:
Preface
Acronyms
Chapter 1: Fitting Potential-Energy Hypersurfaces
Chapter 2: Overview of Some Non-NN Methods for Fitting Ab Initio Potential Energy Databases
Chapter 3: Feed-forward Neural Networks
Chapter 4: Configuration Space Sampling Methods
Chapter 5: Applications of NN Fitting of Potential-Energy Surfaces
Chapter 6: Potential Surfaces Using Expansion Methods and Neural Networks
Chapter 7: Genetic Algorithm (GA) and Internal Energy Transfer Calculations using NN Methods
Chapter 8: Empirical PES Fitting Using Feed-forward Neural Networks
Chapter 9: NN Methods for Data Analysis and Statistical Error Reduction
Chapter 10: Other Applications of NNs to Quantum Mechanical Problems
Chapter 11: Summary, Conclusions, and Future Trends
References
Subject Index