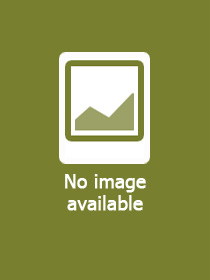
ISBN13: | 9780443248344 |
ISBN10: | 0443248346 |
Binding: | Paperback |
No. of pages: | 250 pages |
Size: | 235x191 mm |
Language: | English |
700 |
Probability for Deep Learning Quantum
EUR 167.99
Click here to subscribe.
Probability for Deep Learning Quantum provides readers with the first book to address probabilistic methods in the deep learning environment and the quantum technological area simultaneously, by using a common platform: the Many-Sorted Algebra (MSA) view. While machine learning is created with a foundation of probability, probability is at the heart of quantum physics as well. It is the cornerstone in quantum applications. These applications include quantum measuring, quantum information theory, quantum communication theory, quantum sensing, quantum signal processing, quantum computing, quantum cryptography, and quantum machine learning. Although some of the probabilistic methods differ in machine learning disciplines from those in the quantum technologies, many techniques are very similar.
Probability is introduced in the text rigorously, in Komogorov’s vision. It is however, slightly modified by developing the theory in a Many-Sorted Algebra setting. This algebraic construct is also used in showing the shared structures underlying much of both machine learning and quantum theory. Both deep learning and quantum technologies have several probabilistic and stochastic methods in common. These methods are described and illustrated using numerous examples within the text. Concepts in entropy are provided from a Shannon as well as a von-Neumann view. Singular value decomposition is applied in machine learning as a basic tool and presented in the Schmidt decomposition. Besides the in-common methods, Born’s rule as well as positive operator valued measures are described and illustrated, along with quasi-probabilities. Author Charles R. Giardina provides clear and concise explanations, accompanied by insightful and thought-provoking visualizations, to deepen your understanding and enable you to apply the concepts to real-world scenarios.
- Provides readers with a resource that is loaded with hundreds of well-crafted examples illustrating the difficult concepts pertaining to quantum and stochastic processes
- Addresses probabilistic methods in the deep learning environment and in the quantum technological area
- Includes a rigorous and precise presentation of the algebraic underpinning of both quantum and deep learning
2. Information geometry
3. Symplectic tomographic probability
4. Born’s rule for quantum probability calculations
5. Msa view for a random variable algebra
6. Algebra illustrations using probability indicators
7. Algebras for complex and quaternion RV
8. Msa for stochastic processes and large deviation theory
9. Probability in canonicle commutational relations
10. Applied probability in quantum
11. Entanglement
12. Quasi probability
13. Noisy Intermediate Scale Quantum NISQ Computing
14. Machine Learning Meets Quantum