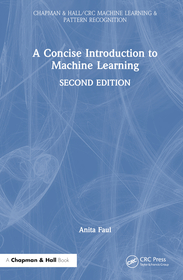
A Concise Introduction to Machine Learning
Sorozatcím: Chapman & Hall/CRC Machine Learning & Pattern Recognition;
-
10% KEDVEZMÉNY?
- A kedvezmény csak az 'Értesítés a kedvenc témákról' hírlevelünk címzettjeinek rendeléseire érvényes.
- Kiadói listaár GBP 130.00
-
Az ár azért becsült, mert a rendelés pillanatában nem lehet pontosan tudni, hogy a beérkezéskor milyen lesz a forint árfolyama az adott termék eredeti devizájához képest. Ha a forint romlana, kissé többet, ha javulna, kissé kevesebbet kell majd fizetnie.
- Kedvezmény(ek) 10% (cc. 6 579 Ft off)
- Discounted price 59 214 Ft (56 394 Ft + 5% áfa)
65 793 Ft
Beszerezhetőség
Még nem jelent meg, de rendelhető. A megjelenéstől számított néhány héten belül megérkezik.
Why don't you give exact delivery time?
A beszerzés időigényét az eddigi tapasztalatokra alapozva adjuk meg. Azért becsült, mert a terméket külföldről hozzuk be, így a kiadó kiszolgálásának pillanatnyi gyorsaságától is függ. A megadottnál gyorsabb és lassabb szállítás is elképzelhető, de mindent megteszünk, hogy Ön a lehető leghamarabb jusson hozzá a termékhez.
A termék adatai:
- Kiadás sorszáma 2
- Kiadó Chapman and Hall
- Megjelenés dátuma 2025. május 14.
- ISBN 9781032878171
- Kötéstípus Keménykötés
- Terjedelem352 oldal
- Méret 234x156 mm
- Nyelv angol
- Illusztrációk 42 Illustrations, black & white; 67 Illustrations, color; 4 Halftones, color; 42 Line drawings, black & white; 63 Line drawings, color; 15 Tables, black & white 700
Kategóriák
Rövid leírás:
A Concise Introduction to Machine Learning uses mathematics as the common language to explain a variety of machine learning concepts from basic principles and illustrates every concept using examples in both Python and MATLAB?, which are available on GitHub and can be run from there in Binder in a web browser.
TöbbHosszú leírás:
A Concise Introduction to Machine Learning uses mathematics as the common language to explain a variety of machine learning concepts from basic principles and illustrates every concept using examples in both Python and MATLAB?, which are available on GitHub and can be run from there in Binder in a web browser. Each chapter concludes with exercises to explore the content.
The emphasis of the book is on the question of Why?only if ?why? an algorithm is successful is understood, can it be properly applied and the results trusted. Standard techniques are treated rigorously, including an introduction to the necessary probability theory. This book addresses the commonalities of methods, aims to give a thorough and in-depth treatment and develop intuition for the inner workings of algorithms, while remaining concise.
This useful reference should be essential on the bookshelf of anyone employing machine learning techniques, since it is born out of strong experience in university teaching and research on algorithms, while remaining approachable and readable.
?Artificial intelligence technologies will be transformational for our society, but not in the manner that the confident proclaimers of utopia and dystopia suggest. The changes we expect are sociotechnical; they require careful understanding from both the societal and technical perspectives. This is where Anita Faul's A Concise Introduction to Machine Learning comes in. With comprehensive coverage of the mathematical principles of machine learning, Anita's book provides the foundation of principled choices that students need to make good decisions when separating the noise from the signal. This foundation is critical to ensuring that we develop the beneficial aspects of the technology while curtailing the damaging outcomes. This can only occur through comprehensive understanding of the capabilities and limitations of these techniques.
Welcome to Anita?s world: a concise introduction to machine learning that builds on solid foundations, practical real-world experience and a long experience of sharing these principles with students.?
- Neil Lawrence, The DeepMind Professor of Machine Learning, University of Cambridge. Senior AI Fellow, The Alan Turing Institute. Visiting Professor of Machine Learning, University of Sheffield
TöbbTartalomjegyzék:
Chapter 1. Introduction
Chapter 2. Probability Theory
Chapter 3. Sampling
Chapter 4. Linear Classification
Chapter 5. Non-Linear Classification
Chapter 6. Dimensionality Reduction
Chapter 7. Regression
Chapter 8. Feature Learning
Appendix A. Matrix Formulae
Index
Több