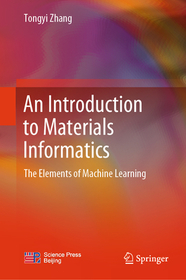
ISBN13: | 9789819979912 |
ISBN10: | 9819979919 |
Kötéstípus: | Keménykötés |
Terjedelem: | 479 oldal |
Méret: | 235x155 mm |
Nyelv: | angol |
Illusztrációk: | 11 Illustrations, black & white; 126 Illustrations, color |
700 |
An Introduction to Materials Informatics
EUR 106.99
Kattintson ide a feliratkozáshoz
A Prosperónál jelenleg nincsen raktáron.
This textbook educates current and future materials workers, engineers, and researchers on Materials Informatics. Volume I serves as an introduction, merging AI, ML, materials science, and engineering. It covers essential topics and algorithms in 11 chapters, including Linear Regression, Neural Networks, and more. Suitable for diverse fields like materials science, physics, and chemistry, it enables quick and easy learning of Materials Informatics for readers without prior AI and ML knowledge.
This textbook educates current and future materials workers, engineers, and researchers on Materials Informatics. Volume I serves as an introduction, merging AI, ML, materials science, and engineering. It covers essential topics and algorithms in 11 chapters, including Linear Regression, Neural Networks, and more. Suitable for diverse fields like materials science, physics, and chemistry, it enables quick and easy learning of Materials Informatics for readers without prior AI and ML knowledge.
Introduction.- Linear Regression.- Linear Classification.- Support Vector Machine.- Decision Tree and K-Nearest-Neighbors (KNN).- Ensemble Learning.- Bayesian Theorem and Expectation-Maximization (EM) Algorithm.- Symbolic Regression.- Neural Networks.- Hidden Markov Chains.- Data Preprocessing and Feature Selection.- Interpretative SHAP Value and Partial Dependence Plot.