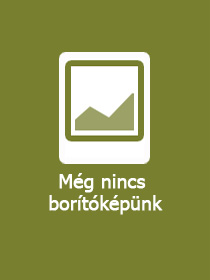
Continual and Reinforcement Learning for Edge AI
Framework, Foundation, and Algorithm Design
Sorozatcím: Synthesis Lectures on Learning, Networks, and Algorithms;
-
8% KEDVEZMÉNY?
- A kedvezmény csak az 'Értesítés a kedvenc témákról' hírlevelünk címzettjeinek rendeléseire érvényes.
- Kiadói listaár EUR 42.79
-
Az ár azért becsült, mert a rendelés pillanatában nem lehet pontosan tudni, hogy a beérkezéskor milyen lesz a forint árfolyama az adott termék eredeti devizájához képest. Ha a forint romlana, kissé többet, ha javulna, kissé kevesebbet kell majd fizetnie.
- Kedvezmény(ek) 8% (cc. 1 452 Ft off)
- Discounted price 16 699 Ft (15 904 Ft + 5% áfa)
18 151 Ft
Beszerezhetőség
Még nem jelent meg, de rendelhető. A megjelenéstől számított néhány héten belül megérkezik.
Why don't you give exact delivery time?
A beszerzés időigényét az eddigi tapasztalatokra alapozva adjuk meg. Azért becsült, mert a terméket külföldről hozzuk be, így a kiadó kiszolgálásának pillanatnyi gyorsaságától is függ. A megadottnál gyorsabb és lassabb szállítás is elképzelhető, de mindent megteszünk, hogy Ön a lehető leghamarabb jusson hozzá a termékhez.
A termék adatai:
- Kiadó Springer
- Megjelenés dátuma 2025. június 17.
- Kötetek száma 1 pieces, Book
- ISBN 9783031843624
- Kötéstípus Keménykötés
- Terjedelem274 oldal
- Méret 240x168 mm
- Nyelv angol
- Illusztrációk 2 Illustrations, black & white; 44 Illustrations, color 700
Kategóriák
Rövid leírás:
This book provides a comprehensive introduction to continual and reinforcement learning for edge AI, which investigates how to build an AI agent that can continuously solve new learning tasks and enhance the AI at resource-limited edge devices. The authors introduce readers to practical frameworks and in-depth algorithmic foundations. The book surveys the recent advances in the area, coming from both academic researchers and industry professionals. The authors also present their own research findings on continual and reinforcement learning for edge AI. The book also covers the practical applications of the topic and identifies exciting future research opportunities.
In addition, this book:
- Provides readers with a deep understanding of the topic, including the framework, foundations, and recent advances
- Discusses the applications of continual and reinforcement learning for edge AI, emphasizing its importance
- Familiarizes readers with the current development stage of the topic in order to inspire further research
About the Authors
Hang Wang is a Ph.D. candidate in the Department of Electrical and Computer Engineering at the University of California, Davis. He received his B.E. from the University of Science and Technology of China (USTC).
Sen Lin, Ph.D., is an Assistant Professor in the Department of Computer Science at University of Houston. He received his Ph.D. degree from Arizona State University, M.S. from HKUST and B.E. from Zhejiang University.
Junshan Zhang, Ph.D. is a Professor in the ECE Department at the University of California, Davis. He received his Ph.D. from the School of ECE at Purdue University.
TöbbHosszú leírás:
This book provides a comprehensive introduction to continual and reinforcement learning for edge AI, which investigates how to build an AI agent that can continuously solve new learning tasks and enhance the AI at resource-limited edge devices. The authors introduce readers to practical frameworks and in-depth algorithmic foundations. The book surveys the recent advances in the area, coming from both academic researchers and industry professionals. The authors also present their own research findings on continual and reinforcement learning for edge AI. The book also covers the practical applications of the topic and identifies exciting future research opportunities.
TöbbTartalomjegyzék:
Introduction to Continual and Reinforcement Learning for Edge AI.- Algorithmic and Theoretical Foundations.- Federated Continual Learning.- On-device Continual Learning.- Online Meta-Learning.- Warm-start Reinforcement Learning.- Continual Reinforcement Learning.- Continual and Reinforcement Learning for Edge AI with Pre-trained Large Language Models.
Több