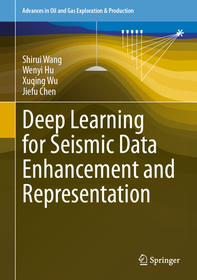
ISBN13: | 9783031757440 |
ISBN10: | 30317574411 |
Kötéstípus: | Keménykötés |
Terjedelem: | 152 oldal |
Méret: | 254x178 mm |
Nyelv: | angol |
Illusztrációk: | 27 Illustrations, black & white; 80 Illustrations, color |
700 |
Deep Learning for Seismic Data Enhancement and Representation
EUR 181.89
Kattintson ide a feliratkozáshoz
Seismic imaging is a key component of subsurface exploration, and it depends on a high-quality seismic data acquisition system with effective seismic processing algorithms. Seismic data quality concerns various factors such as acquisition design, environmental constraints, sampling resolution, and noises. The focus of this book is to investigate efficient seismic data representation and signal enhancement solutions by leveraging the powerful feature engineering capability of deep learning.
The book delves into seismic data representation and enhancement issues, ranging from seismic acquisition design to subsequent quality improvement and compression technologies. Given the challenges of obtaining suitable labeled training datasets for seismic data processing problems, we concentrate on exploring deep learning approaches that eliminate the need for labels. We combined novel deep learning techniques with conventional seismic data processing methods, and construct networks and frameworks tailored for seismic data processing. The editors and authors of this book come from both academia and industry with hands-on experiences in seismic data processing and imaging.
Seismic imaging is a key component of subsurface exploration, and it depends on a high-quality seismic data acquisition system with effective seismic processing algorithms. Seismic data quality concerns various factors such as acquisition design, environmental constraints, sampling resolution, and noises. The focus of this book is to investigate efficient seismic data representation and signal enhancement solutions by leveraging the powerful feature engineering capability of deep learning.
The book delves into seismic data representation and enhancement issues, ranging from seismic acquisition design to subsequent quality improvement and compression technologies. Given the challenges of obtaining suitable labeled training datasets for seismic data processing problems, we concentrate on exploring deep learning approaches that eliminate the need for labels. We combined novel deep learning techniques with conventional seismic data processing methods, and construct networks and frameworks tailored for seismic data processing. The editors and authors of this book come from both academia and industry with hands-on experiences in seismic data processing and imaging.
Chapter 1: Introduction.- Chapter 2: Full Waveform Inversion With Low-Frequency Extrapolation.- 3: Deep Learning For Seismic Deblending.- Chapter 4: Blind-Trace Network For Self-Supervised Seismic Data Interpolation.- Chapter 5: Self-Supervised Learning For Anti-Aliased Seismic Data Interpolation Using Dip Information.- Chapter 6:Deep Learning For Seismic Data Compression.- Chapter 7: Conclusion.