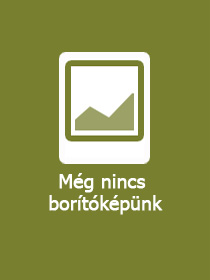
ISBN13: | 9780443236419 |
ISBN10: | 04432364111 |
Kötéstípus: | Puhakötés |
Terjedelem: | 230 oldal |
Méret: | 234x190 mm |
Súly: | 450 g |
Nyelv: | angol |
700 |
Federated Learning for Medical Imaging
EUR 128.00
Kattintson ide a feliratkozáshoz
Federated Learning for Medical Imaging: Principles, Algorithms, and Applications gives a deep understanding of the technology of federated learning (FL), the architecture of a federated system, and the algorithms for FL. It shows how FL allows multiple medical institutes to collaboratively train and use a precise machine learning (ML) model without sharing private medical data via practical implantation guidance. The book includes real-world case studies and applications of FL, demonstrating how this technology can be used to solve complex problems in medical imaging. The book also provides an understanding of the challenges and limitations of FL for medical imaging, including issues related to data and device heterogeneity, privacy concerns, synchronization and communication, etc.
This book is a complete resource for computer scientists and engineers, as well as clinicians and medical care policy makers, wanting to learn about the application of federated learning to medical imaging.
Section I Fundamentals of FL
1. Background
2. FL Foundations
Section II Advanced Concepts and Methods for Heterogenous Settings
3. FL on Heterogeneous Data
4. FL on long-tail (label)
5. Personalized FL
6. Cross-domain FL
Section III Trustworthy FL
7. FL and Fairness
8. Differential Privacy
9. Security (Attack and Defense) in FL
10. FL + Uncertainty
11. Noisy learning in FL
Section IV Real-world Implementation and Application
12. Image Segmentation
13. Image Reconstruction and Registration
14. Frameworks and Platforms
Section V Afterword
15. Summary and Outlook