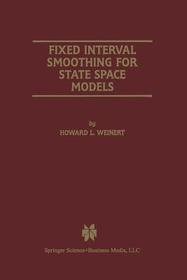
A termék adatai:
ISBN13: | 9781461356806 |
ISBN10: | 1461356806 |
Kötéstípus: | Puhakötés |
Terjedelem: | 119 oldal |
Méret: | 235x155 mm |
Súly: | 215 g |
Nyelv: | angol |
Illusztrációk: | X, 119 p. |
0 |
Témakör:
Fixed Interval Smoothing for State Space Models
Kiadás sorszáma: Softcover reprint of the original 1st ed. 2001
Kiadó: Springer
Megjelenés dátuma: 2012. október 24.
Kötetek száma: 1 pieces, Book
Normál ár:
Kiadói listaár:
EUR 106.99
EUR 106.99
Az Ön ára:
41 753 (39 765 Ft + 5% áfa )
Kedvezmény(ek): 8% (kb. 3 631 Ft)
A kedvezmény csak az 'Értesítés a kedvenc témákról' hírlevelünk címzettjeinek rendeléseire érvényes.
Kattintson ide a feliratkozáshoz
Kattintson ide a feliratkozáshoz
Beszerezhetőség:
Becsült beszerzési idő: A Prosperónál jelenleg nincsen raktáron, de a kiadónál igen. Beszerzés kb. 3-5 hét..
A Prosperónál jelenleg nincsen raktáron.
Nem tudnak pontosabbat?
A Prosperónál jelenleg nincsen raktáron.
Hosszú leírás:
Fixed-interval smoothing is a method of extracting useful information from inaccurate data. It has been applied to problems in engineering, the physical sciences, and the social sciences, in areas such as control, communications, signal processing, acoustics, geophysics, oceanography, statistics, econometrics, and structural analysis.
This monograph addresses problems for which a linear stochastic state space model is available, in which case the objective is to compute the linear least-squares estimate of the state vector in a fixed interval, using observations previously collected in that interval. The author uses a geometric approach based on the method of complementary models. Using the simplest possible notation, he presents straightforward derivations of the four types of fixed-interval smoothing algorithms, and compares the algorithms in terms of efficiency and applicability. Results show that the best algorithm has received the least attention in the literature.
Fixed Interval Smoothing for State Space Models:
`In the reviewer's opinion, this monograph is pioneering in a fascinating and relatively new field of research. It should prove useful to people working in control theory and doing research on smoothing, and for those who want to choose a smoothing algorithm for a particular application.'
Zdzislaw W. Trzaska, American Mathematical Society
Springer Book Archives
This monograph addresses problems for which a linear stochastic state space model is available, in which case the objective is to compute the linear least-squares estimate of the state vector in a fixed interval, using observations previously collected in that interval. The author uses a geometric approach based on the method of complementary models. Using the simplest possible notation, he presents straightforward derivations of the four types of fixed-interval smoothing algorithms, and compares the algorithms in terms of efficiency and applicability. Results show that the best algorithm has received the least attention in the literature.
Fixed Interval Smoothing for State Space Models:
- includes new material on interpolation, fast square root implementations, and boundary value models;
- is the first book devoted to smoothing;
- contains an annotated bibliography of smoothing literature;
- uses simple notation and clear derivations;
- compares algorithms from a computational perspective;
- identifies a best algorithm.
`In the reviewer's opinion, this monograph is pioneering in a fascinating and relatively new field of research. It should prove useful to people working in control theory and doing research on smoothing, and for those who want to choose a smoothing algorithm for a particular application.'
Zdzislaw W. Trzaska, American Mathematical Society
Springer Book Archives
Tartalomjegyzék:
Ch. 1 Introduction.- 1.1 State Space Models.- 1.2 Fixed Interval Smoothing.- 1.3 Notes and References.- Ch. 2 Complementary Models.- 2.1 Discrete Case.- 2.2 Continuous Case.- 2.3 Notes and References.- Ch. 3 Discrete Smoothers.- 3.1 Backward-Forward Smoother.- 3.2 Forward-Backward Smoothers.- 3.3 Two-Filter Smoother.- 3.4 Square Root Implementations.- 3.5 Interpolated Case.- 3.6 Notes and References.- Ch. 4 Continuous Smoothers.- 4.1 Backward-Forward Smoother.- 4.2 Forward-Backward Smoothers.- 4.3 Two-Filter Smoother.- 4.4 Notes and References.- Ch. 5 Boundary Value Models.- 5.1 Complementary Model.- 5.2 Backward-Forward Smoother.- 5.3 Notes and References.- Annotated Bibliography.- Author Index.