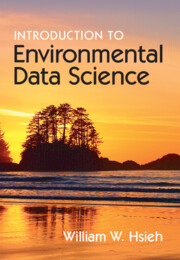
A termék adatai:
ISBN13: | 9781107065550 |
ISBN10: | 11070655511 |
Kötéstípus: | Keménykötés |
Terjedelem: | 647 oldal |
Méret: | 250x175x35 mm |
Súly: | 1330 g |
Nyelv: | angol |
539 |
Témakör:
Adatbázis kezelő szoftverek
Kiegészítő eszközök
Adatelemzés
Mesterséges intelligencia
Környezetmérnöki tudományok
További könyvek a számítástechnika területén
Környezetvédelem
Meteorológia
További könyvek a földtudományok területén
Környezettudomány általában
Adatbázis kezelő szoftverek (karitatív célú kampány)
Kiegészítő eszközök (karitatív célú kampány)
Adatelemzés (karitatív célú kampány)
Mesterséges intelligencia (karitatív célú kampány)
Környezetmérnöki tudományok (karitatív célú kampány)
További könyvek a számítástechnika területén (karitatív célú kampány)
Környezetvédelem (karitatív célú kampány)
Meteorológia (karitatív célú kampány)
További könyvek a földtudományok területén (karitatív célú kampány)
Környezettudomány általában (karitatív célú kampány)
Introduction to Environmental Data Science
Kiadó: Cambridge University Press
Megjelenés dátuma: 2023. március 23.
Normál ár:
Kiadói listaár:
GBP 59.99
GBP 59.99
Az Ön ára:
24 541 (23 372 Ft + 5% áfa )
Kedvezmény(ek): 20% (kb. 6 135 Ft)
A kedvezmény érvényes eddig: 2024. december 31.
A kedvezmény csak az 'Értesítés a kedvenc témákról' hírlevelünk címzettjeinek rendeléseire érvényes.
Kattintson ide a feliratkozáshoz
Kattintson ide a feliratkozáshoz
Beszerezhetőség:
Becsült beszerzési idő: A Prosperónál jelenleg nincsen raktáron, de a kiadónál igen. Beszerzés kb. 3-5 hét..
A Prosperónál jelenleg nincsen raktáron.
Nem tudnak pontosabbat?
A Prosperónál jelenleg nincsen raktáron.
Rövid leírás:
A comprehensive guide to machine learning and statistics for students and researchers of environmental data science.
Hosszú leírás:
Statistical and machine learning methods have many applications in the environmental sciences, including prediction and data analysis in meteorology, hydrology and oceanography; pattern recognition for satellite images from remote sensing; management of agriculture and forests; assessment of climate change; and much more. With rapid advances in machine learning in the last decade, this book provides an urgently needed, comprehensive guide to machine learning and statistics for students and researchers interested in environmental data science. It includes intuitive explanations covering the relevant background mathematics, with examples drawn from the environmental sciences. A broad range of topics is covered, including correlation, regression, classification, clustering, neural networks, random forests, boosting, kernel methods, evolutionary algorithms and deep learning, as well as the recent merging of machine learning and physics. End&&&8209;of&&&8209;chapter exercises allow readers to develop their problem-solving skills, and online datasets allow readers to practise analysis of real data.
'As a new wave of machine learning becomes part of our toolbox for environmental science, this book is both a guide to the latest developments and a comprehensive textbook on statistics and data science.&&&160;Almost everything is covered, from hypothesis testing to convolutional neural networks.&&&160;The book is enjoyable to read, well explained and economically written, so it will probably become the first place I'll go to read up on any of these topics.' Alan Geer, European Centre for Medium-Range Weather Forecasts (ECMWF)
'As a new wave of machine learning becomes part of our toolbox for environmental science, this book is both a guide to the latest developments and a comprehensive textbook on statistics and data science.&&&160;Almost everything is covered, from hypothesis testing to convolutional neural networks.&&&160;The book is enjoyable to read, well explained and economically written, so it will probably become the first place I'll go to read up on any of these topics.' Alan Geer, European Centre for Medium-Range Weather Forecasts (ECMWF)
Tartalomjegyzék:
1. Introduction; 2. Basics; 3. Probability distributions; 4. Statistical inference; 5. Linear regression; 6. Neural networks; 7. Nonlinear optimization; 8. Learning and generalization; 9. Principal components and canonical correlation; 10. Unsupervised learning; 11. Time series; 12. Classification; 13. Kernel methods; 14. Decision trees, random forests and boosting; 15. Deep learning; 16. Forecast verification and post-processing; 17. Merging of machine learning and physics; Appendices; References; Index.