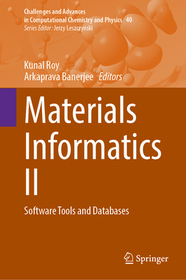
ISBN13: | 9783031787270 |
ISBN10: | 3031787277 |
Kötéstípus: | Keménykötés |
Terjedelem: | 297 oldal |
Méret: | 235x155 mm |
Nyelv: | angol |
Illusztrációk: | 7 Illustrations, black & white; 95 Illustrations, color |
700 |
Materials Informatics II
EUR 213.99
Kattintson ide a feliratkozáshoz
This contributed volume explores the application of machine learning in predictive modeling within the fields of materials science, nanotechnology, and cheminformatics. It covers a range of topics, including electronic properties of metal nanoclusters, carbon quantum dots, toxicity assessments of nanomaterials, and predictive modeling for fullerenes and perovskite materials. Additionally, the book discusses multiscale modeling and advanced decision support systems for nanomaterial risk management, while also highlighting various machine learning tools, databases, and web platforms designed to predict the properties of materials and molecules. It is a comprehensive guide and a great tool for researchers working at the intersection of machine learning and material sciences.
This contributed volume explores the application of machine learning in predictive modeling within the fields of materials science, nanotechnology, and cheminformatics. It covers a range of topics, including electronic properties of metal nanoclusters, carbon quantum dots, toxicity assessments of nanomaterials, and predictive modeling for fullerenes and perovskite materials. Additionally, the book discusses multiscale modeling and advanced decision support systems for nanomaterial risk management, while also highlighting various machine learning tools, databases, and web platforms designed to predict the properties of materials and molecules. It is a comprehensive guide and a great tool for researchers working at the intersection of machine learning and material sciences.
Part 1. Introduction.- Introduction to Machine Learning for Predictive Modeling I.- Introduction to Machine Learning for Materials Property Modeling.- Part 2. Cheminformatic and Machine Learning Models for Nanomaterials.- Machine learning models to study electronic properties of metal nanoclusters.- Applications of Machine Learning Predictive Modeling for Carbon Quantum Dots.- Assessing the toxicity of quantum dots in healthy and tumoral cells with ProtoNANO, a platform of nano-QSAR models to predict the toxicity of inorganic nanomaterials.- Applications of predictive modeling for fullerenes.- Computational Analysis of Perovskite Materials AlXY3 (X = Cu, Mn; Y = Br, Cl, F) invoking the DFT Method.- Applications of predictive modeling for dye-sensitized solar cells (DSSCs).- Introduction to multiscale modeling for One Health approaches.- DIAGONAL Decision Support System (DSS) for Advanced Nanomaterial Risk Management powered by Enalos Cloud Platform.- Part 3. Software Tools and Databases for Applications in Materials Science.- Machine Learning algorithms, tools, and databases for applications in Materials Science.- Machine Learning-Driven Web Tools for Predicting Properties of Materials and Molecules.