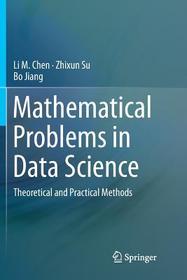
Mathematical Problems in Data Science
Theoretical and Practical Methods
-
8% KEDVEZMÉNY?
- A kedvezmény csak az 'Értesítés a kedvenc témákról' hírlevelünk címzettjeinek rendeléseire érvényes.
- Kiadói listaár EUR 128.39
-
Az ár azért becsült, mert a rendelés pillanatában nem lehet pontosan tudni, hogy a beérkezéskor milyen lesz a forint árfolyama az adott termék eredeti devizájához képest. Ha a forint romlana, kissé többet, ha javulna, kissé kevesebbet kell majd fizetnie.
- Kedvezmény(ek) 8% (cc. 4 357 Ft off)
- Discounted price 50 105 Ft (47 719 Ft + 5% áfa)
54 463 Ft
Beszerezhetőség
Becsült beszerzési idő: A Prosperónál jelenleg nincsen raktáron, de a kiadónál igen. Beszerzés kb. 3-5 hét..
A Prosperónál jelenleg nincsen raktáron.
Why don't you give exact delivery time?
A beszerzés időigényét az eddigi tapasztalatokra alapozva adjuk meg. Azért becsült, mert a terméket külföldről hozzuk be, így a kiadó kiszolgálásának pillanatnyi gyorsaságától is függ. A megadottnál gyorsabb és lassabb szállítás is elképzelhető, de mindent megteszünk, hogy Ön a lehető leghamarabb jusson hozzá a termékhez.
A termék adatai:
- Kiadás sorszáma Softcover reprint of the original 1st ed. 2015
- Kiadó Springer
- Megjelenés dátuma 2019. március 14.
- Kötetek száma 1 pieces, Previously published in hardcover
- ISBN 9783319797397
- Kötéstípus Puhakötés
- Terjedelem213 oldal
- Méret 235x155 mm
- Súly 454 g
- Nyelv angol
- Illusztrációk 22 Illustrations, black & white; 42 Illustrations, color 3
Kategóriák
Hosszú leírás:
This book describes current problems in data science and Big Data. Key topics are data classification, Graph Cut, the Laplacian Matrix, Google Page Rank, efficient algorithms, hardness of problems, different types of big data, geometric data structures, topological data processing, and various learning methods. For unsolved problems such as incomplete data relation and reconstruction, the book includes possible solutions and both statistical and computational methods for data analysis. Initial chapters focus on exploring the properties of incomplete data sets and partial-connectedness among data points or data sets. Discussions also cover the completion problem of Netflix matrix; machine learning method on massive data sets; image segmentation and video search. This book introduces software tools for data science and Big Data such MapReduce, Hadoop, and Spark.
This book contains three parts. The first part explores the fundamental tools of data science. It includes basic graph theoretical methods, statistical and AI methods for massive data sets. In second part, chapters focus on the procedural treatment of data science problems including machine learning methods, mathematical image and video processing, topological data analysis, and statistical methods. The final section provides case studies on special topics in variational learning, manifold learning, business and financial data rec
overy, geometric search, and computing models.Mathematical Problems in Data Science is a valuable resource for researchers and professionals working in data science, information systems and networks. Advanced-level students studying computer science, electrical engineering and mathematics will also find the content helpful.
?Data science includes mathematical and statistical tools required to find relations and principles behind heterogeneous and possibly unstructured data. It is an emerging field, under active research, and the authors here have attempted to explain existing methods whole introducing some open problems. ? Overall, the book offers a collection of papers that describe current trends and future directions along with appropriate references. The presented applications cover a broad spectrum of domains where big data poses challenges.? (Paparao Kayalipati, Computing Reviews, computingreviews.com, September, 2016)
TöbbTartalomjegyzék:
Introduction: Data Science and BigData Computing.- Overview of Basic Methods for Data Science.- Relationship and Connectivity of Incomplete Data Collection.- Machine Learning for Data Science: Mathematical or Computational.- Images, Videos, and BigData.- Topological Data Analysis.- Monte Carlo Methods and their Applications in Big Data Analysis.- Feature Extraction via Vector Bundle Learning.- Curve Interpolation and Financial Curve Construction.- Advanced Methods in Variational Learning: Segmentation with Intensity Inhomogeneity.- An On-line Strategy of Groups Evacuation From a Convex Region in the Plane.- A New Computational Model of Bigdata.
Több