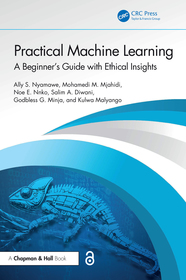
ISBN13: | 9781032770291 |
ISBN10: | 1032770295 |
Kötéstípus: | Puhakötés |
Terjedelem: | 192 oldal |
Méret: | 234x156 mm |
Nyelv: | angol |
Illusztrációk: | 55 Illustrations, black & white; 20 Illustrations, color; 4 Halftones, black & white; 5 Halftones, color; 51 Line drawings, black & white; 15 Line drawings, color; 43 Tables, black & white |
700 |
Villamosmérnöki tudományok, híradástechnika, műszeripar
Szoftverfejlesztés
Mesterséges intelligencia
Programnyelvek általában
Környezetmérnöki tudományok
Villamosmérnöki tudományok, híradástechnika, műszeripar (karitatív célú kampány)
Szoftverfejlesztés (karitatív célú kampány)
Mesterséges intelligencia (karitatív célú kampány)
Programnyelvek általában (karitatív célú kampány)
Környezetmérnöki tudományok (karitatív célú kampány)
Practical Machine Learning
GBP 48.99
Kattintson ide a feliratkozáshoz
This is a core resource for students and instructors of machine learning and data science looking for beginner-friendly material which offers real-world applications and takes ethical discussions into account.
The book provides an accessible, comprehensive introduction for beginners to machine learning, equipping them with the fundamental skills and techniques essential for this field.
It enables beginners to construct practical, real-world solutions powered by machine learning across diverse application domains. It demonstrates the fundamental techniques involved in data collection, integration, cleansing, transformation, development, and deployment of machine learning models. This book emphasizes the importance of integrating responsible and explainable AI into machine learning models, ensuring these principles are prioritized rather than treated as an afterthought. To support learning, this book also offers information on accessing additional machine learning resources such as datasets, libraries, pre-trained models, and tools for tracking machine learning models.
This is a core resource for students and instructors of machine learning and data science looking for beginner-friendly material which offers real-world applications and takes ethical discussions into account.
1. Fundamentals of Machine Learning 2. Mathematics for Machine Learning 3. Data Preparation 4. Machine Learning Operations 5. Machine Learning Software and Hardware Requirements 6. Responsible AI and Explainable AI 7. Artificial General Intelligence 8. Machine Learning Step-by-Step Practical Examples