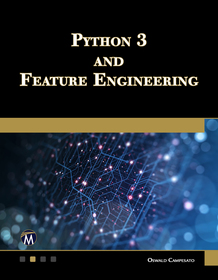
A termék adatai:
ISBN13: | 9781683929499 |
ISBN10: | 1683929497 |
Kötéstípus: | Puhakötés |
Terjedelem: | 216 oldal |
Méret: | 0x0x0 mm |
Súly: | 326 g |
Nyelv: | angol |
590 |
Témakör:
Python 3 and Feature Engineering
Kiadás sorszáma: 1
Kiadó: Mercury Learning and Information
Megjelenés dátuma: 2023. december 27.
Kötetek száma: Großformatiges Paperback. Klappenbroschur
Normál ár:
Kiadói listaár:
EUR 50.00
EUR 50.00
Az Ön ára:
20 648 (19 665 Ft + 5% áfa )
Kedvezmény(ek): 5% (kb. 1 087 Ft)
A kedvezmény csak az 'Értesítés a kedvenc témákról' hírlevelünk címzettjeinek rendeléseire érvényes.
Kattintson ide a feliratkozáshoz
Kattintson ide a feliratkozáshoz
Beszerezhetőség:
Megrendelésre a kiadó utánnyomja a könyvet. Rendelhető, de a szokásosnál kicsit lassabban érkezik meg.
Nem tudnak pontosabbat?
Hosszú leírás:
This book is designed for data scientists, machine learning practitioners, and anyone with a foundational understanding of Python 3.x. In the evolving field of data science, the ability to manipulate and understand datasets is crucial. The book offers content for mastering these skills using Python 3. The book provides a fast-paced introduction to a wealth of feature engineering concepts, equipping readers with the knowledge needed to transform raw data into meaningful information. Inside, you?ll find a detailed exploration of various types of data, methodologies for outlier detection using Scikit-Learn, strategies for robust data cleaning, and the intricacies of data wrangling. The book further explores feature selection, detailing methods for handling imbalanced datasets, and gives a practical overview of feature engineering, including scaling and extraction techniques necessary for different machine learning algorithms. It concludes with a treatment of dimensionality reduction, where you?ll navigate through complex concepts like PCA and various reduction techniques, with an emphasis on the powerful Scikit-Learn framework.