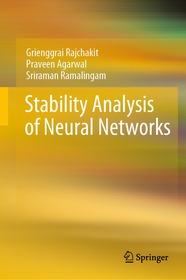
ISBN13: | 9789811665332 |
ISBN10: | 9811665338 |
Kötéstípus: | Keménykötés |
Terjedelem: | 404 oldal |
Méret: | 235x155 mm |
Súly: | 811 g |
Nyelv: | angol |
Illusztrációk: | 2 Illustrations, black & white; 54 Illustrations, color |
277 |
Stability Analysis of Neural Networks
EUR 139.09
Kattintson ide a feliratkozáshoz
A Prosperónál jelenleg nincsen raktáron.
This book discusses recent research on the stability of various neural networks with constrained signals. It investigates stability problems for delayed dynamical systems where the main purpose of the research is to reduce the conservativeness of the stability criteria. The book mainly focuses on the qualitative stability analysis of continuous-time as well as discrete-time neural networks with delays by presenting the theoretical development and real-life applications in these research areas. The discussed stability concept is in the sense of Lyapunov, and, naturally, the proof method is based on the Lyapunov stability theory. The present book will serve as a guide to enable the reader in pursuing the study of further topics in greater depth and is a valuable reference for young researcher and scientists.
This book discusses recent research on the stability of various neural networks with constrained signals. It investigates stability problems for delayed dynamical systems where the main purpose of the research is to reduce the conservativeness of the stability criteria. The book mainly focuses on the qualitative stability analysis of continuous-time as well as discrete-time neural networks with delays by presenting the theoretical development and real-life applications in these research areas. The discussed stability concept is in the sense of Lyapunov, and, naturally, the proof method is based on the Lyapunov stability theory. The present book will serve as a guide to enable the reader in pursuing the study of further topics in greater depth and is a valuable reference for young researcher and scientists.
?This book presents the foundations of stability analysis of neural networks in a well-organized manner. ? This book will be very helpful to the research community working in the area of stability of dynamical systems, especially neural networks. Readers who are familiar with the basics of differential equations will find it very comfortable.? (Raju K. George, Mathematical Reviews, February, 2023)