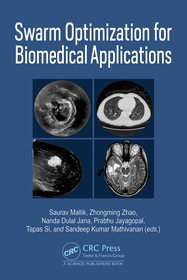
ISBN13: | 9781032697338 |
ISBN10: | 1032697334 |
Kötéstípus: | Keménykötés |
Terjedelem: | 326 oldal |
Méret: | 234x156 mm |
Nyelv: | angol |
Illusztrációk: | 83 Illustrations, black & white; 8 Illustrations, color; 41 Halftones, black & white; 2 Halftones, color; 42 Line drawings, black & white; 6 Line drawings, color |
700 |
Valószínűségelmélet és matematikai statisztika
Optimalizáció, lineáris programozás, játékelmélet
Alkalmazott matematika
A biológia általános kérdései
Energetika, energiaipar
A számítástudomány elmélete, a számítástechnika általában
Mesterséges intelligencia
Digitális jel-, hang- és képfeldolgozás
Orvosi biotechnológia
Swarm Optimization for Biomedical Applications
GBP 140.00
Kattintson ide a feliratkozáshoz
With the recent advancements in machine learning (ML) and deep learning (DL), ML/DL techniques are being widely used in biomedical engineering to develop intelligent decision-making healthcare systems in real-time. Various optimization techniques have been employed to optimize parameters, hyper-parameters.
Biomedical engineering is a rapidly growing interdisciplinary area that is providing solutions to biological and medical problems and improving the healthcare system. It is connected to various applications like protein structure prediction, computer-aided drug design, and computerized medical diagnosis based on image and signal data, which accomplish low-cost, accurate, and reliable solutions for improving healthcare services. With the recent advancements, machine learning (ML) and deep learning (DL) techniques are widely used in biomedical engineering to develop intelligent decision-making healthcare systems in real-time. However, accuracy and reliability in model performance can be a concern in tackling data generated from medical images and signals, making it challenging for researchers and practitioners. Therefore, optimized models can produce quality healthcare services to handle the complexities involved in biomedical research.
Various optimization techniques have been employed to optimize parameters, hyper-parameters, and architectural information of ML/DL models explicitly applied to biological, medical, and signal data. The swarm intelligence approach has the potential to solve complex non-linear optimization problems. It mimics the collective behavior of social swarms such as ant colonies, honey bees, and bird flocks. The cooperative nature of swarms can search global settings of ML/DL models, which efficiently provide the solution to biomedical engineering applications. Finally, the book aims to provide the utility of swarm optimization and similar optimization techniques to design ML/DL models to improve the solutions related to biomedical engineering.